Poster Abstracts
O 01 Khadija Aichane-Simon
E 02 Marius Amann
O 02 Varvara Apet
E 03 Katharina Bachschwöller
E 04 Anna Backeberg
A 01 Julian Beck
E 05 Philipp Becker
O 03 Stine Behrmann
P 01 Ramachandra M Bhaskara
O 04 Patryk Bielski
A 02 Sophie Binder
A 03 Andreas Blaha
A 04 Alexander Braun
A 05 Stephan Breimann
O 05 Constanze Breithaupt
E 07 Adrian Bunzel
P 02 Grzegorz Chojnowski
P 03 Sandrine Coquille
O 06 Jan A. Dewald
O 07 Karthikeyan Dhamotharan
M 01 Simon Dürr
A 06 Stefan Düsterhöft
O 08 Christoph Elfmann
O 09 Mohammad ElGamacy
A 07 Robert Englmeier
M 02 Inken Fender
O 10 Anna Franziska Finke
O 11 Karlo Franke
E 01 Emily Freund
O 12 Béla Frohn
O 13 Andreas Gagsteiger
A 08 Chiara Gasbarri
O 14 Florian Gisdon
E 37 Anastasia Götz
E 08 Salomé Guilbert
M 03 Jannik Adrian Gut
O 15 Jan Hartmann
E 09 Noah Ernst Holzleitner
E 10 Stefan Hristov
A 09 Maxim Janzen
E 11 Michael Adrian Jendrusch
E 12 Alena Khmelinskaia
E 13 Hubert Klein
A 10 Sarah Knapp
E 14 Johanna-Sophie Koch
E 15 Kerlen Korbeld
P 04 Jacqueline Krohn
E 16 Georg Künze
P 05 Pasquale Lamagna
O 16 Olive Laprévote
E 17 Horst Lechner
T 01 Jennifer Leclaire
E 18 Bruce Lichtenstein
P 06 Katja Luck
O 17 Marharyta Makarova
A 11 Yelyzaveta Makedon
P 07 Anja Marquardt
E 20 David Medina-Ortiz
P 08 Pasquale Miglionico
A 12 Johanna Moeller
A 13 Felix Moorhoff
E 21 Lennart Nickel
E 22 Martin Pacesa
O 18 Marina Parr
O 19 Lars-oliver Peters
A 14 Thorsten Pfirrmann
O 20 Agata Raczyńska
P 10 René Rasche
O 21 Johanna Rathke
E 23 Katharina Rauchenwald-Köchl
O 22 Johannes Regner
P 11 Bianca Reschke
P 12 Till Rudack
T 02 Jakob Ruickoldt
P 13 Peter Russ
E 24 Marc Scherer
E 25 Phillip Schlegel
E 26 Lisa Schmidt
T 03 Clara Schoeder
M 04 Leif Seute
A 15 Irina Shevyreva
O 23 Ingrid Span
P 15 Simon Straß
P 16 Milton T. Stubbs
E 27 Andrey Sysoev
P 17 Tomer Tsaban
O 24 Pascal Vielberth
E 28 Vsevolod Viliuga
E 29 Leo von Bank
O 25 Srdan Vujinovic
E 30 Simon Wagner
A 16 Daniel Wedemeyer
E 31 Jonas Wepfer
E 32 Timothy Whitehead
O 26 Nex Maris Winkler
E 33 Benedict Wolf
E 34 Tobias Wörtwein
E 35 Christina Wüst
M 06 Max Dongsheng Yin
P 14 Büsra Yüksel
E 36 Cathleen Zeymer
M 05 Hanne Zillmer
E 19 Anna Zink
A 17 Aleksandr Zlobin
A 01 Julian Beck
A 02 Sophie Binder
A 03 Andreas Blaha
A 04 Alexander Braun
A 05 Stephan Breimann
A 06 Stefan Düsterhöft
A 07 Robert Englmeier
A 08 Chiara Gasbarri
A 09 Maxim Janzen
A 10 Sarah Knapp
A 11 Yelyzaveta Makedon
A 12 Johanna Moeller
A 13 Felix Moorhoff
A 14 Thorsten Pfirrmann
A 15 Irina Shevyreva
A 16 Daniel Wedemeyer
A 17 Aleksandr Zlobin
E 01 Emily Freund
E 02 Marius Amann
E 03 Katharina Bachschwöller
E 04 Anna Backeberg
E 05 Philipp Becker
E 07 Adrian Bunzel
E 08 Salomé Guilbert
E 09 Noah Ernst Holzleitner
E 10 Stefan Hristov
E 11 Michael Adrian Jendrusch
E 12 Alena Khmelinskaia
E 13 Hubert Klein
E 14 Johanna-Sophie Koch
E 15 Kerlen Korbeld
E 16 Georg Künze
E 17 Horst Lechner
E 18 Bruce Lichtenstein
E 19 Anna Zink
E 20 David Medina-Ortiz
E 21 Lennart Nickel
E 22 Martin Pacesa
E 23 Katharina Rauchenwald-Köchl
E 24 Marc Scherer
E 25 Phillip Schlegel
E 26 Lisa Schmidt
E 27 Andrey Sysoev
E 28 Vsevolod Viliuga
E 29 Leo von Bank
E 30 Simon Wagner
E 31 Jonas Wepfer
E 32 Timothy Whitehead
E 33 Benedict Wolf
E 34 Tobias Wörtwein
E 35 Christina Wüst
E 36 Cathleen Zeymer
E 37 Anastasia Götz
M 01 Simon Dürr
M 02 Inken Fender
M 03 Jannik Adrian Gut
M 04 Leif Seute
M 05 Hanne Zillmer
M 06 Max Dongsheng Yin
O 01 Khadija Aichane-Simon
O 02 Varvara Apet
O 03 Stine Behrmann
O 04 Patryk Bielski
O 05 Constanze Breithaupt
O 06 Jan A. Dewald
O 07 Karthikeyan Dhamotharan
O 08 Christoph Elfmann
O 09 Mohammad ElGamacy
O 10 Anna Franziska Finke
O 11 Karlo Franke
O 12 Béla Frohn
O 13 Andreas Gagsteiger
O 14 Florian Gisdon
O 15 Jan Hartmann
O 16 Olive Laprévote
O 17 Marharyta Makarova
O 18 Marina Parr
O 19 Lars-oliver Peters
O 20 Agata Raczyńska
O 21 Johanna Rathke
O 22 Johannes Regner
O 23 Ingrid Span
O 24 Pascal Vielberth
O 25 Srdan Vujinovic
O 26 Nex Maris Winkler
P 01 Ramachandra M Bhaskara
P 02 Grzegorz Chojnowski
P 03 Sandrine Coquille
P 04 Jacqueline Krohn
P 05 Pasquale Lamagna
P 06 Katja Luck
P 07 Anja Marquardt
P 08 Pasquale Miglionico
P 10 René Rasche
P 11 Bianca Reschke
P 12 Till Rudack
P 13 Peter Russ
P 14 Büsra Yüksel
P 15 Simon Straß
P 16 Milton T. Stubbs
P 17 Tomer Tsaban
T 01 Jennifer Leclaire
T 02 Jakob Ruickoldt
T 03 Clara Schoeder
A 01
Scaffolding a Kemp eliminase activity into an idealized de novo TIM barrel
Presenter: Julian Beck (julian.beck@uni-bayreuth.de)
Authors:
Julian Beck¹; Benjamin Smith²; Emily Freund¹; Niayesh Zarifi²; Roberto Chica²; Birte Höcker¹
¹ University of Bayreuth; ² University of Ottawa, Canada
The ubiquitous (βα)8- or TIM-barrel fold is adopted by 10% of all known enzymes and present in six out of seven Enzyme Commission classes. Due to its success in nature, the TIM-barrel fold is a highly interesting target for enzyme design. After decades of attempts the first de novo TIM barrel with a highly idealized four-fold symmetric topology and minimal loops was designed in 2016. Despite this milestone and multiple optimizations de novo TIM barrels remained inactive as they lack structural features for substrate binding and catalysis based on their minimalistic architecture. Here, we present CANVAS, a computational workflow to introduce structural extensions to anchor catalytic residues and form an active-site pocket for enzymatic activity. The multistep design workflow combines physics- and AI-based tools like Triad and RFdiffusion and is used to introduce a Kemp eliminase activity into an idealized de novo TIM barrel. Experimental characterization identified an active design with catalytic efficiency comparable to previously reported computationally designed de novo Kemp eliminases. The developed CANVAS workflow, which resulted in the first enzymatically active de novo TIM barrel, provides a generalizable platform to utilize the full enzymatic potential of de novo TIM barrels and other scaffolds in the future.
_________________
A 02
AI-based protein design and validation
Presenter: Sophie Binder (sobind@mailbox.org)
Authors:
Sophie Binder¹; Bianca Broske²; Benjamin A. McEnroe²; Marie Kleinert²; Dominic Ferber¹; Julia Meßmer²; Elisabeth Tan²; Tim N. Kempchen²; Caroline I. Fandrey³; Alexander Hoch³; Peter Konopka³; Katja Blumenstock³; Prof. Dr. Matthias Geyer¹; Prof. Dr. Jonathan Schmid-Burgk³; Dr. Stephan Menzel⁴; Dr. Gregor Hagelueken¹; Prof. Dr. Michael Hoelzel²
¹ Institute of Structural Biology, University of Bonn; ² Institute of Experimental Oncology, University of Bonn; ³ Institute of Clinical Chemistry and Clinical Pharmacology, University of Bonn; ⁴ Core Facility Nanobodies, University of Bonn
AI-based prediction of protein structures is a very recent and rapidly evolving topic with high potential in the field of cancer research and development of novel therapeutic agents, in particular in settings of difficult antibody or nanobody production.
Using alpha-fold-based prediction algorithms, we generated libraries of artificial proteins designed to bind specific target structures. Through a cellular screening system, we identified a subset of binders that were validated to successfully bind their respective targets. Binding to target proteins was verified in FACS experiments using cellular systems as well as recombinantly expressed proteins. When studying the binding kinetics using SPR spectroscopy, we observed high binding affinities in the nanomolar range. Further, the AI-Binders exhibited a high thermal stability and a notable ability to refold after denaturation.
The AI-Binders are readily expressed in E. coli and can be easily purified to homogeneity. Preliminary crystallization trials revealed that the AI-Binders are highly inclined to form crystals, enabling their use as crystallization chaperones. By site-specific biotinylation and functionalization with streptavidin, we generated tetravalent target-binding scaffolds, which are promising tools for target protein structure determination using cryo-electron microscopy.
_________________
A 03
A conserved fertilization complex predicted by AlphaFold2-Multimer bridges sperm and egg in vertebrates
Presenter: Andreas Blaha (andreas.blaha@live.de)
Authors:
Victoria Deneke; Andreas Blaha; Andrea Pauli
Research Institute of Molecular Pathology (IMP), Vienna, Austria
Fertilization, the basis for sexual reproduction, culminates in the binding and fusion of sperm and egg. Although several proteins are known to be crucial for this process in vertebrates, the molecular mechanisms remain poorly understood. Using an AlphaFold-Multimer screen, we identified the protein Tmem81 as part of a conserved trimeric sperm complex with the essential fertilization factors Izumo1 and Spaca6. We demonstrate that Tmem81 is essential for male fertility in zebrafish and mice. In line with trimer formation, we show that Izumo1, Spaca6, and Tmem81 interact in zebrafish sperm and that the human orthologs interact in vitro. Notably, complex formation creates the binding site for the egg fertilization factor Bouncer in zebrafish. Together, our work presents an compelling example how AI-driven structure and interaction prediction leads to a comprehensive model in basic life science. Namely, a model for fertilization across vertebrates, where a conserved sperm complex binds to divergent egg proteins—Bouncer in fish and JUNO in mammals—to mediate sperm-egg interaction.
_________________
A 04
De novo design of triosephosphate isomerases using generative language models
Presenter: Alexander Braun (alexander.e.braun@uni-bayreuth.de)
Authors:
Alexander Braun
University of Bayreuth
The design of proteins with tailored function is of immense interest to biotechnology, medicine, and the chemical industry. While protein design is rapidly evolving with the use of AI techniques, the design of complex enzymes remains a challenge. Here, we explore the use of two large language models (LLMs), the conditional ZymCTRL and the unconditional ProtGPT2, for the generation of de novo triosephosphate isomerases (TIM). These enzymes adopt obligatory oligomers, catalyze the multistep interconversion reaction near the diffusion limit, and are well-researched. These attributes make this enzyme class the optimal model for investigating the ability of different protein LLMs to recreate structure, function, and protein-protein interactions on the primary structure level. Multiple constructs from both language models exhibit the intended function in vivo through their ability to complement a TIM-deficient E. coli strain. In-depth characterization of the best-behaving artificial TIM reveals behavior and catalytic efficiency close to its natural counterparts. Further, we investigate the role of sequence parameters in the training dataset, the influence of activity-independent filtering on the sequence performance, and the ability of protein LLMs to recreate intra-protein interactions throughout the length of the sequence. Our findings support the use of conditional and fine-tuned unconditional LLMs for the generation of complex enzymes.
_________________
A 05
Charting γ-Secretase substrates by explainable AI
Presenter: Stephan Breimann (stephan.breimann@dzne.de)
Authors:
Stephan Breimann¹; Prof. Dr. Harald Steiner²; Prof. Dr. Dmitrij Frishman¹; Dr. Frits Kamp³
¹ Technical University of Munich; ² Ludwig-Maximilians-University Munich (LMU); ³ Ludwigs-Maximilians Universität München
Proteases recognize substrates by decoding sequence information, yet this remains elusive without clear motifs. We tackled this for γ-secretase, a key intramembrane protease in Alzheimer’s and cancer, by developing Comparative Physicochemical Profiling (CPP), a sequence-based algorithm for interpretable feature engineering. CPP identified a γ-secretase substrate signature based on discriminative physicochemical properties at specific residues. To address dataset imbalance, we introduced dPULearn, a deterministic positive-unlabeled learning algorithm. Using machine learning, we ranked CPP features and predicted the γ-secretase substrate proteome with 90% accuracy—outperforming protein language models (~60%)—and validated predictions experimentally (88% success rate). Integrating CPP with SHAP enabled explaining prediction results at single-residue resolution. Our work advances interpretable machine learning-based protein prediction and protein design.
_________________
A 06
MaPIN: A computational pipeline to Map Protein Interaction Networks.
Presenter: Dr. Stefan Düsterhöft (sduesterhoeft@ukaachen.de)
Authors:
Katharina Schun; Sarah Knapp; Dr. Stefan Düsterhöft
University Hospital Aachen
Multiomics is advancing our understanding of gene regulation and expression, but often obscures crucial molecular and functional details, such as protein interactions and signalling networks. Experimentally determining each interaction is laborious, highlighting the need for automated solutions.
We have developed MaPIN, a high-throughput pipeline that integrates extensive experimental proteomics/interactomics data and AlphaFold-based interaction prediction with transcriptomic datasets, such as single-cell RNA-seq, to identify, score and prioritise molecular targets and networks for experimental investigation.
We validated MaPIN using the iRhom-ADAM17 complex, a transmembrane protease centrally involved in signalling pathways essential for development and immune responses. Precise control of its activity and localisation is critical; loss of function impairs immune responses, while dysregulation is associated with chronic inflammation, carcinogenesis and viral infections. Here, MaPIN revealed novel modes of interactions with this complex, including a non-canonical, phosphorylation-independent interaction with 14-3-3 proteins.
In summary, MaPIN provides an automated, high-throughput framework for mapping protein networks and signalling pathways. As a key analysis tool for proteomics and interactomics data, MaPIN can facilitate the identification of complex pathophysiological networks and new drug targets to modify pathological processes.
_________________
A 07
Towards automating cryo-EM data analysis using AI, new algorithms and large scale analysis
Presenter: Robert Englmeier PhD (robert@cryocloud.io)
Authors:
Gydo van Zundert PhD; Anna Boronina; Robert Englmeier PhD
CryoCloud
In the last decade, improved algorithms have facilitated structure determination by cryo-EM routinely yielding ~3 Å resolutions for optimized and well-behaved samples,. However, challenging samples that display compositional or conformational heterogeneity, or preferred particle orientation, often require extensive optimization and complex data analysis protocols. These datasets are characterized by repeated particle localization, extensive classifications or anisotropy, making data analysis time-intensive and manual. Here, we present developments to overcome challenges of difficult samples: a new ML based particle picker for fast and accurate particle localisation, as well as algorithms to analyze map anisotropy. Our picker shows improved precision and faster performance compared to current pickers. Importantly, it gives improved results without the need of parameter inputs or optimizations across a range of datasets. To quantify anisotropy from preferred particle orientation, we have implemented new algorithms for analyzing anisotropy. Applying them at large scale to >15,000 maps from the EMDB reliably and objectively identified maps that suffer from anisotropy, which can be computationally corrected in some cases. Combined, these tools facilitate data analysis, enable automation across a wider range of datasets, and provide an improved quantitative readout for anisotropy that can objectively guide and improve cryo-EM data analysis and sample optimization.
_________________
A 08
AI in support of drug off-target effect research: the CavitOmix Copilot
Presenter: Chiara Gasbarri (chiara.gasbarri@innophore.com)
Authors:
Chiara Gasbarri; Katharina Rauchenwald-Köchl; Lena Parigger; Haris Rudalija; Michael Hetmann; Markus Fleck; Tobias Schopper; Georg Steinkellner; Christian C. Gruber
Innophore GmbH
Safety concerns account for approximately 17% of failures in phase 3 clinical trials, often resulting from therapeutic agents binding to unintended protein targets with similar binding pockets to the desired targets. Predicting similarities between protein pockets could help reduce the failure rate in drug discovery programs. To address this issue, we introduce CavitOmiX Copilot, a novel platform developed by Innophore, which employs an AI-based matching approach to identify structural similarities between protein pockets.
Through comprehensive modeling of the human proteome using homology modeling and advanced AI techniques, we generate multidimensional point clouds known as CatalophoresTM. These point clouds represent a fingerprint of protein binding pockets by encoding their physicochemical properties, which are subsequently embedded into high-dimensional vectors.
This framework facilitates ultra-rapid comparisons and matching of different cavities, allowing for the detection of similarities between proteins that are not biased by protein sequence or structure. Consequently, potential binding sites that might otherwise remain unexplored are revealed. CavitOmix Copilot is available as a web application at https://cavitomix.catalophore.com/.
_________________
A 09
Predicting single residue effects on peptide binding specificities in designed Armadillo repeat proteins
Presenter: Maxim Janzen (maxim-janzen@gmx.de)
Authors:
Jakob Noske; Maxim Janzen; Prof. Dr. Birte Höcker
University of Bayreuth
The PreART (Predictive Reagent Antibody Replacement Technology) project aims to replace existing reagent antibodies by a peptide-binding system based on designed Armadillo repeat proteins (dArmRP). A major challenge in this process is the design of highly specific single amino acid binding pockets. Since experimentally determining binding affinities is expensive and time-consuming, there is a need for accurate in silico scoring methods. While physics-based scoring functions such as BBK*, Flex ddG, and PocketOptimizer can predict some effects, they also show biases towards specific amino acids. Here, we tested combining protein structure prediction with affinity scoring via the Poisson-Boltzmann Surface Area method and observed significant improvements in the prediction of dArmRP binding affinities and specificity. The major advantages of this method are, on one hand, the use of Chai-1, which enables structure predictions without the need for a template or extensive modeling, and on the other hand, the Poisson-Boltzmann calculations, which provide a highly precise method for calculating electrostatic energies.
_________________
A 10
Predicting PARP15 networks with proximity-ligation based mass spectrometry data and MaPIN (Mapping of Protein Interaction Networks)
Presenter: Sarah Knapp (sarah.knapp@rwth-aachen.de)
Authors:
Sarah Knapp; Lukas Hauser; Prof. Bernhard Lüscher; Dr. Stefan Düsterhöft; Dr. Patricia Korn
University Hospital Aachen, RWTH Aachen University
Mono-ADP-ribosylation (MARylation) plays a key role in various cellular processes, including transcriptional regulation, host-pathogen interactions, and cellular stress responses. PARP15, a protein involved in MARylation, remains poorly characterised in terms of its physiological and pathophysiological functions. To explore its interaction partners and associated molecular networks, we generated stable U2OS Flp-In™ T-REx™ cells expressing the V5-miniTurbo-PARP15 fusion protein upon induction. Biotinylated proteins were enriched and analysed using mass spectrometry. Comparing the results to a catalytically inactive mutant of PARP15 we aimed to identify potential substrates of PARP15 mediated protein MARylation.
To predict protein interaction networks and identify specific interaction sites, we employed MaPIN, a novel high-throughput pipeline that integrates experimental proteomics and interactomics data with AlphaFold-based interaction predictions. MaPIN provides a powerful tool for mapping protein interactions and signalling pathways. Furthermore, it allows to narrow down specific protein-interaction sites. For PARP15 we combine data from our proximity-labelling mass spectrometry screen with data from public databases such as BioGRID. This approach enables us to identify, score, and prioritise molecular targets and networks for further experimental validation.
_________________
A 11
The role of Beta-2 microglobulin tryptophan mutagenesis in detecting MHC class I heavy chain folding in vitro
Presenter: Yelyzaveta Makedon (ymakedon@constructor.university)
Authors:
Yelyzaveta Makedon; Prof. Dr. Sebastian Springer
Jacobs University Bremen gGmbH/ Constructor University Bremen gGmbH
Recombinant major histocompatibility complex (MHC) class I proteins, folded in vitro, are used as MHC tetramers to identify antigen-specific T cells for immune monitoring, epitope discovery, and T cell isolation. To rapidly assess different folding scenarios in small volumes, nanoscale differential scanning fluorimetry (NanoDSF) can be used to monitor thermal stability through tryptophan fluorescence. The challenge arises that in such setups, the melting temperature (Tm) of the folded complexes of MHC-I heavy chain (HC) and light chain (beta-2 microglobulin, β2m) complexes is close to that of the folded β2m alone, making measurements difficult. Thus, we mutagenized tryptophan residues 60 and 95 of β2m to phenylalanine. To analyse potential structural changes at a theoretical level, we used AlphaFold2 and DynaMut before initiating wet lab experiments. A comparison of the structures generated by both tools revealed no significant differences, reinforcing the reliability of these predictions in experimental design. Employing these β2m mutants in a complex with an HC allows for the separate detection of the Tm of the folded HC. The results elucidate the role of β2m tryptophans in the fluorescent signal detected by NanoDSF and enhance our understanding of MHC-I HC folding dynamics with different peptides.
_________________
A 12
Computational stabilization and functional characterization of single-chain T cell receptors targeting a cancer epitope
Presenter: Johanna Moeller (johanna.moeller@uni-leipzig.de)
Authors:
Johanna Moeller¹; Max Alexander Lingner Chango²; Victoria Most²; Clara Tabea Schoeder²
¹ Center for Scalable Data Analytics and Artificial Intelligence (ScaDS.AI) Dresden/Leipzig; ² Faculty of Medicine, Leipzig University
Advances in the development of T cell-based therapeutics have led to an increased interest in the T cell receptor (TCR) and its natural ligand, the peptide loaded major histocompatibility complex (pMHC). TCRs are heterodimeric, complicating their handling and application as therapeutics. Thus, single-chain TCR (scTv) constructs would be highly beneficial. Here, we apply rational protein design to increase stability of the highly unstable scTvs. Mutants were expressed in vitro and evaluated for stability as well as functionality.
The known TCR 1G4 (targeting NY-ESO1 peptide bound to HLA-A*0201) was chosen as a template. The scTv was rationally designed using ProteinMPNN, a deep learning-based sequence design method (Dauparas J et al., 2022), Rosetta, a software-suite with a physics- and statistics-based energy function (Kuhlman B, 2019) and PROSS, a phylogenetically guided design approach (Goldenzweig A et al., 2016). The resultant mutants were expressed and tested for thermostability and functionality.
Computational protein design of the chosen scTv led to improved expression rates and higher thermostability compared to wild type. The measured affinities of scTvs agreed with KD values known from literature, confirming the functionality of the stabilized scTvs. In conclusion, we introduced a pipeline for the computational stabilization and expression of pMHC-binders, thus facilitating the streamlined design and optimization of specific T-cell epitope binders.
_________________
A 13
SelectZyme: AI-Based Interactive Protein Space Visualization for Enzyme Discovery, Selection, and Mining
Presenter: Felix Moorhoff (fmoorhof@ipb-halle.de)
Authors:
Felix Moorhoff; Dr. David Alfredo Medina-Ortiz PhD; Dr. Martin Dippe PhD; Dr. Mehdi D. Davari PhD; Prof. Dr. Ludger A. Wessjohann PhD
Leibniz Institute of Plant Biochemistry (IPB)
Enzymes are pivotal in biotechnology. Despite their importance, most enzymes remain uncharacterized, obscuring their potential value for biocatalysis. Enzyme mining address this challenge by leveraging the sequence-structure-function relationship. However, existing tools face significant limitations, including labor-intensive manual parameter tuning, difficulties in visualization, and inaccurate cluster representations, often from alignment-based methods that struggle with sequence variability in length and homology. Protein language models offer representations with proven success in enzyme mining. Yet, their complexity limits accessibility for non-expert users. This work introduces SelectZyme, an embedding-based enzyme mining tool for effective navigation of large enzyme latent spaces. SelectZyme simplifies enzyme selection, requiring only query terms to initiate analysis. Integrated clustering and dimensionality reduction generate interactive visualizations, protein landscape plots, phylogenetic trees, and a minimal spanning tree. Besides, SelectZyme tracks user selections and outputs annotated tables of sequences of interest. To demonstrate its utility, we applied SelectZyme to three datasets addressing key challenges in biocatalysis. By streamlining enzyme mining and visualization, SelectZyme empowers researchers to unlock the functional and biocatalytic potential of uncharacterized enzymes, accelerating advancements in biotechnology for users of all expertise levels.
_________________
A 14
CiliAI: A Web-Based Machine Learning Platform for Automated Detection, Analysis, and Signal Densitometry of Primary Cilia in Confocal Microscopy Images
Presenter: Prof. Dr. Thorsten Pfirrmann (thorsten.pfirrmann@hmu-potsdam.de)
Authors:
Prof. Dr. Thorsten Pfirrmann¹; Dr. Christoph Gerhardt¹; Eliza Karapetian¹; Emrah Nazif²
¹ Health and Medical University Potsdam; ² Datamarkin
Primary cilia are cellular organelles that sense extracellular signals through membrane-localized receptors, regulating key processes like cell proliferation, differentiation, polarity, and development. Defects in primary cilia cause ciliopathies, a diverse group of disorders affecting multiple organs and leading to developmental abnormalities, sensory impairments, and complex clinical manifestations. Traditional methods for cilia analysis, such as immunofluorescence microscopy and manual image processing, are labor intensive and time consuming. Here, we introduce CiliAI, a machine learning-based platform designed to automate the detection and analysis of primary cilia from confocal microscopy images. CiliAI is designed to measure various parameters, including cilia length, number of ciliated cells, and protein densitometry, with high accuracy and speed. Our web-based tool employs multiple AI models and a series of algorithms to analyze 3D image arrays, detect cilia in each z-stack, and assess specific ciliary substructures. The platform generates detailed reports within minutes, significantly enhancing the precision and rapidity of primary cilia analysis. CiliAI holds promise of advancement in primary cilia research, contributing for the study of ciliary biology and the diagnosis of ciliopathies and related disorders.
_________________
A 15
Understanding the catalytic mechanism of bacterial phospholipid N-methyltransferases using AI tools and not only
Presenter: Irina Shevyreva (irina.shevyreva@ruhr-uni-bochum.de)
Authors:
Irina Shevyreva¹; Dr. Meriyem Aktas; Prof. Dr. Franz Narberhaus
¹ Ruhr university Bochum
Phosphatidylcholine (PC), a typical eukaryotic phospholipid, is an important compound of some bacterial membranes. In certain bacteria, PC plays a crucial role in facilitating interactions with their eukaryotic hosts. One of the common PC biosynthesis routes in bacteria is a three-step methylation of phosphatidylethanolamine mediated by phospholipid N-methyltransferases (Pmts). Pmts are classified into the Sinorhizobium (S) and Rhodobacter (R) types. Despite extensive biochemical and structural studies of the S-type Agrobacterium tumefaciens PmtA (AtPmtA) and the R-type Rubellimicrobium thermophilum (RtPmtA), understanding of the catalytic mechanism in bacterial Pmts remains insufficient.
The main goal of this project was the elucidation and comparative analysis of the catalytic mechanisms of S-type and R-type Pmts. For this purpose, we employed a combination of computational and biochemical research.
We suggest that the two Pmt classes exhibit similar reaction mechanisms with tyrosine residues mainly involved in the catalysis of methyl group transfer. RtPmtA features two highly conserved tyrosines in the substrate-binding pocket, located on the N-terminal αA-helix. In the S-type AtPmtA, only one tyrosine, positioned in the protein core, has an impact on the enzyme´s activity. Furthermore, we propose that the specific active site modifications indicate distinct mechanisms for tyrosine activation, thereby illustrating the evolutionary divergence of the two Pmt types.
_________________
A 16
Protein half-lives and destabilizing regions via language models
Presenter: Daniel Wedemeyer (daniel.wedemeyer@uni-hamburg.de)
Authors:
Daniel Wedemeyer¹; Prof. Andrew E. Torda²
¹ Universität Hamburg ; ² Universität Hamburg
Cellular protein homeostasis reflects both protein production and degradation. E3 ubiquitin ligases direct proteasomal degradation through ubiquitination in a substrate-structure-dependent manner by binding so-called degron motifs. Intracellular half-lives of human proteins have been measured through isotope labelling and mass spectrometry. While these methods enable high-throughput measurement of intracellular proteins, the experiments are expensive and labour-intensive.
We curated a comprehensive dataset from the human proteome from published data, revealing consistency of relative degradation rates between cell types independently of differences in global protein turnover. We trained a protein language model to predict intracellular half-lives based on protein sequence. Model generalizability was ensured by partitioning training and test data based on sequence similarity. Our model achieved a Spearman correlation of 0.65 for proteins with not more than 40% sequence identity to any protein of the training set. By masking parts of input sequences, one can identify destabilizing regions. These sometimes agree with known degrons, but sometimes suggest new possible degrons.
Predicting the impact of subtle sequence differences on half-lives may enable tuning the degradation of engineered proteins for therapeutic and technical applications.
_________________
A 17
Towards robust computational activity assays for enzyme study and design
Presenter: Dr. Aleksandr Zlobin (aleksandr.zlobin@medizin.uni-leipzig.de)
Authors:
Dr. Aleksandr Zlobin; Prof. Dr. Jens Meiler
Leipzig University
Computational enzyme design is a promising technique for producing novel enzymes for industrial and clinical needs. A key challenge this technique faces is to consistently achieve the desired activity. Some limitations come from the inherent inaccuracies of scoring functions (e.g. in Rosetta), while others from the reductionist approaches for design. We hypothesized that supplementing computational enzyme design pipelines with reaction modeling-derived barrier estimation could increase success rates and yield fundamental insights.
A reaction modeling-based approach for design should be fast, automatized, transferable, and report fair uncertainties of barrier estimates that should be low. We achieve all these requirements with our approach. At the core of it are data-learned (AI) reaction coordinates that power dynamical sampling of the reaction process in hybrid QM/MM simulations. We show that these variables are general enough for one set once trained to be able to model reactions in any Ser-His-Asp/Glu triad hydrolases. Applied to the case of protease Subtilisin, our approach helped uncover a crucial role of distant charged residues in achieving native activity – an insight for consideration in de novo enzyme design. We are looking forward to using our approach as an in silico assay within de novo enzyme design pipelines, screening hundreds of candidate variants on their way to experimental validation.
_________________
E 01
Redesigned RNA-binding domains to study their effects on helicases
Presenter: Emily Freund (Emily.Freund@uni-bayreuth.de)
Authors:
Emily Freund; Nina Lang; Dr. Kristian Schweimer; Prof. Dr. Janosch Hennig
University of Bayreuth
NTP-dependent helicases are essential for unwinding RNA or DNA, playing a crucial role in cell viability. Given their functional importance, helicases contribute to genomic stability and regulate key stages of the RNA cycle. In addition to interacting with RNA, helicases also engage with various proteins within their environment or through their auxiliary domains. These interactions are often critical for the localization of RNA targets or for regulating helicase activity. Therefore, understanding not only the RNA-binding properties of helicases but also their protein-protein interactions is essential.
To investigate protein-protein interactions of helicases, we developed a computational design pipeline that facilitates the rapid design of soluble and stable domains. These domains are derived from well-characterized RNA-binding domains but lack RNA-binding functionality. Since RNA-binding domains are highly conserved across different helicases, their sequence identities can be leveraged for redesign. This approach provides a valuable tool for studying their effects on helicase activity and cellular RNA-related processes, as it preserves protein-protein interactions while eliminating RNA-binding capability. In this work, we biochemically characterized a redesigned RNA-binding domain using diverse methods, including NMR and helicase assays.
_________________
E 02
Engineering Plasmodium falciparum Proteins for Small-Molecule Drug Discovery: Investigating Stability, Structural Dynamics, and Biophysical Effects
Presenter: Marius Amann (marius.amann@pharmazie.uni-freiburg.de)
Authors:
Marius Amann; Tim Strässer; Prof. Dr. Oliver Einsle; Prof. Dr. Stefan Günther
Albert Ludwigs Universität Freiburg
To facilitate drug discovery targeting Plasmodium falciparum proteins, we applied ProteinMPNN-driven mutagenesis to enhance their stability and expression in E. coli, enabling purification, crystallization, and structural characterization. We used isothermal titration calorimetry (ITC) to study the biophysical properties of wild-type and ProteinMPNN-optimized variants within a drug discovery pipeline. Unexpectedly, in one of the proteins, X-ray crystallography revealed a loop-to-helix transition outside the mutated regions—an effect not predicted by AlphaFold—induced by the designed mutations. To further explore these structural dynamics, we are using various computational methods, ranging from protein language models to molecular dynamics simulations, to pinpoint the mutations driving this conformational change.
_________________
E 03
Design of functional proteins by fragment-based chimeragenesis
Presenter: Katharina Bachschwöller (katharina.bachschwoeller@uni-bayreuth.de)
Authors:
Katharina Bachschwöller
University of Bayreuth
Protein domains are considered the fundamental units of protein structure, function and evolution. They act as building blocks that play an important role in the diversity of the protein universe. Domains are thought to have evolved from smaller ancestral fragments that have been repurposed within protein folds across different structural and functional contexts, leading to shared conserved fragments. As such, the recombination of these fragments holds the potential to generate proteins with novel functions, presenting a starting point for rational protein design.
To test this hypothesis, the chimeric protein TyrAFld was constructed. It comprises an ATP-binding fragment derived from a Rossmann-fold domain recombined with a fragment of an FMN-binding flavodoxin-like domain. The spatial arrangement of both ligands within the model suggests that TyrAFld could potentially catalyze the formation of FAD. Due to previous results indicating mostly insolubly expressed protein, the sequence of the chimera was redesigned whilst conserving the ligand binding sites utilizing different methods, including PROSS, ProteinMPNN and LigandMPNN.
Most of these newly designed TyrAFld variants are expressed soluble and in high yields. Biochemical characterization indicates correct folding and high thermal stability. Preliminary experimental ITC data indicates ATP binding. Further experimental binding analysis has to be conducted.
_________________
E 04
De novo design of multiple-geometry forming protein scaffolds
Presenter: Anna Backeberg (Anna.Backeberg@campus.lmu.de)
Authors:
Anna Backeberg; Elizaveta Maltseva; Ho Yeung Chim; Prof. Dr. Alena Khmelinskaia
Polyhedral protein assemblies are highly functional materials with applications in areas such as drug-delivery or vaccine development. While the de novo design of static protein nanoparticles with one specific target geometry is well established and exceedingly accurate, this study aims at the design of rigid protein monomers that can simultaneously self-assemble into different geometries. Just like having a versatile ingredient in a kitchen pantry, our goal is to establish "off-the-shelf" programmability of protein assemblies with a set of universal building blocks, facilitating the targeted design of new and functional protein nanoparticles. Combining current Artificial Intelligence (AI)-based design methods with physics-based approaches, we established a pipeline for the design of such multiple-geometry forming protein scaffolds. Our pipeline tackles this challenge on two levels: (i) the generation of backbones matching multiple geometries; and (ii) the design of degenerate sequences compatible with equal formation of the target architectures. Our approach paves the way for a new generation of highly versatile protein building blocks.
_________________
E 05
Past to Protocells: Ancestral Min Proteins and Early Pattern Formation
Presenter: Philipp Becker (pbecker@biochem.mpg.de)
Authors:
Philipp Becker; Béla Frohn; Prof. Dr. Petra Schwille
Max Planck Institute of Biochemistry
Self-organized protein patterns drive many cellular processes, including bacterial cell division. One prominent example is the Min system, in which the ATPase MinD and its activating factor MinE form a reaction-diffusion system that positions the fission ring midcell. Although well-characterized, little is known about its emergence during evolution. Here, we employ ancestral sequence reconstruction (ASR) as a protein-design strategy to resurrect putative MinD and MinE ancestors. While ASR is often used to enhance protein properties, it also reveals key evolutionary events linked to functional innovation. To investigate early steps of pattern formation, we integrate these ancestral proteins into synthetic cells designed to mimic primitive protocells. Altogether, our findings highlight how evolutionary reconstructions, combined with synthetic cells, can shed new light on the origins and diversification of complex cellular machineries.
_________________
E 07
AI.zymes: A Modular Platform for Evolutionary Enzyme Design
Presenter: Dr. Adrian Bunzel (adrian.bunzel@bsse.ethz.ch)
Authors:
Dr. Adrian Bunzel
ETH Zurich
Reliable enzyme design would revolutionize bioengineering, yet computational design approaches often fail to achieve high activity. We address this challenge by combining protein design with directed evolution to create highly active enzymes [1]. By evolving designer enzymes, we have uncovered catalytic limitations in designer enzymes, suggesting how computational algorithms may be improved [2–4].
Protein design algorithms typically focus on stability, restricting their suitability for enzyme design. To overcome this, we developed AI.zymes [6], a modular evolutionary design platform integrating Rosetta, ESMFold, ProteinMPNN, and FieldTools. Iterative design-selection cycles allowed the optimization of properties critical to biocatalysis, like electrostatic catalysis, which the employed design programs do not directly target. AI.zymes was validated by improving the promiscuous Kemp eliminase activity of ketosteroid isomerase 7.7-fold after testing 7 variants.
Reliable enzyme design likely requires a holistic framework that accounts for dynamic and electrostatic biocatalytic effects. AI.zymes meets this need by integrating advanced bioengineering algorithms for the evolutionary design of features specific to enzyme activity.
[1] Bunzel HA et al., J Am Chem Soc, 2019.
[2] Bunzel HA et al., Curr Opin Struct Biol, 2021.
[3] Otten R et al., Science, 2020.
[4] Jabeen H et al., ACS Catal, 2024.
[5] Merlicek LP et al., bioRxiv, 2025.
_________________
E 08
In Silico Design of Enzymes to Degrade Short-chain Per- and Polyfluoroalkyl Substances (PFAS)
Presenter: Salomé Guilbert PhD (salome.guilbert@epfl.ch)
Authors:
Salomé Guilbert PhD¹; Prof. Philippe Schwaller²; Prof. Ursula Roethlisberger²
¹ Ecole Polytechnique Fédérale Lausanne; ² EPFL
Increasing concern over the persistence and toxicity of per- and polyfluoroalkyl substances (PFAS) has pushed for the need to design technologies for their remediation. To date, the only commercially degradation method involves costly and unsustainable thermal deconstruction. Bioremediation stands as an attractive, cost-effective and sustainable solution for degrading such pollutants, although few bacteria and no enzymes are known to achieve the full degradation of PFAS.
The in silico rational design of novel enzymes for PFAS degradation first involved the selection of a suitable biological target that had the potential to be optimised for better substrate binding, catalytic activity and thermal stability. A theozyme was created with the catalytic residues of fluoroacetate dehalogenase, a well-studied enzyme capable of breaking the strong carbon-fluorine bond (460 kJ/mol) in fluoroacetate. First, RFDiffusionAA helped generate a library of suitable scaffolds around the theozyme. After sequence design with ProteinMPNN, AlphaFold was used to filter the sequences that folded in the desired structures.
To assess the catalytic reaction of these enzyme designs, QM/MM simulations will be performed. Further, selected biomimetic systems will be optimised with genetic algorithms, classical molecular simulations and machine learning tools such as AI.Zymes. This process will be applied to short-chain PFAS molecules, often overlooked as degradation products of longer-chain PFAS.
_________________
E 09
AI-guided engineering of CRISPR-Cas proteins
Presenter: Noah Ernst Holzleitner (noah.holzleitner@tum.de)
Authors:
Noah Ernst Holzleitner¹; Dr. Jessica Kornherr¹; Christian Plumeyer; Prof. Dr. Julian Grünewald
¹ TU Munich
The large size of the most commonly used CRISPR-Cas protein, Streptococcus pyogenes Cas9 (SpCas9), challenges in vivo delivery due to the limited packaging capacity of AAVs (~4.7kb). Recently, several alternative miniature Cas proteins were discovered by mining large metagenomic datasets. However, their therapeutic potential is often limited by low gene editing activity in human cells. In this study, we engineer two miniature CRISPR-Cas12L proteins and their crRNAs for enhanced editing in human cells, combining both structure-guided and AI-based strategies. This hybrid approach resulted in up to 400-fold improvement of gene editing activities in human cells. Based on this pipeline and experimental feedback, we aim to generate an AI agent for engineering CRISPR-Cas proteins.
_________________
E 10
ForceYield2: Validating AI-Assisted Protein Design With In Vivo Selection
Presenter: Stefan Hristov (stefan.hristov@charite.de)
Authors:
Stefan Hristov¹; Ehsan Salehabadi¹; Anton Tadilla Stefan Hristov¹; Dr. Beau Dronsella²; Irina Piven¹; Dr. Steffen Lindner Stefan Hristov¹
¹ Charite´- Universitätsmedizin Berlin; ² Max Planck Institute for Terrestrial Microbiology
Anthropogenic climate change has created an urgent need for an emissions-free chemical industry, driving innovations in synthetic biology that enable the creation of microbial cell factories producing sustainable alternatives to fossil-derived compounds. Metabolic engineering now benefits from AI-driven structural biology tools, such as AlphaFold, Foldseek, and RoseTTAFold, which have revolutionized enzyme identification and engineering. These advancements simplify the design of optimized and even new-to-nature proteins. However, validating AI-designed enzymes remains challenging, as current methods rely on moderate-throughput techniques requiring complex workflows and apparatus. In vivo enzyme engineering, which couples enzymatic activity to microbial fitness, offers a promising solution by simplifying workflows and enabling high-throughput screening (Orsi et al., 2024). In this work, glucarate—valued for its applications in biodegradable detergents, biopolymers, and pharmaceuticals—is produced in E. coli via a synthetic glycolysis bypass. The pathway is constrained by the low catalytic efficiency of inositol-1-phosphatase (IPS), necessitating enzyme engineering. We successfully engineered a growth-coupled strain linking IPS function to fitness, allowing direct screening of designed variants via a simple growth readout. This study is proof of concept for the synergy of AI-driven enzyme design and growth-coupled selection, driving innovation toward a circular bioeconomy.
_________________
E 11
Fast and flexible protein structure generation with sparse all-atom denoising (SALAD)
Presenter: Michael Adrian Jendrusch (michael.jendrusch@embl.de)
Authors:
Michael Adrian Jendrusch; Prof. Dr. Jan Korbel
European Molecular Biology Laboratory
Protein backbone generation, sequence design and structure predictor-based filtering form the backbone of the modern machine learning-assisted protein design pipeline. However, current protein backbone generators have to contend with a three-way trade-off between efficiency, designability and flexibility. While comparatively slow, hallucination-based approaches reach high designability even for large proteins and can be flexibly adapted to different protein design tasks merely by changing the fitness function being optimized. Conversely, protein structure diffusion models offer high generation efficiency and designability for small proteins, at the cost of flexibility and designability for large proteins. To address this issue, we introduce a family of sparse all-atom denoising models (salad) for efficient and flexible protein backbone generation. salad addresses efficiency by using sparse attention without persistent amino acid pair features, outperforming prior diffusion models in terms of runtime. Simultaneously, salad bridges the designability gap to hallucination-based methods, reliably designing protein backbones with up to 1,000 amino acids. Finally, by combining salad with structure-editing – a modified generative process for diffusion models – we can adapt it to produce scaffolds for protein motifs, repeat proteins and proteins predicted to adopt multiple conformations under different conditions, bridging the gap in flexibility to hallucination-based methods.
_________________
E 12
Tales of Dynamic Protein Assembly Design
Presenter: Dr. Alena Khmelinskaia (akhmelin@cup.lmu.de)
Authors:
Dr. Alena Khmelinskaia
Ludwig-Maximilians-Universität München
Recent computational methods have been developed for designing novel protein assemblies with atomic-level accuracy, yet several aspects of current methods limit the structural and functional space that can be explored. I will share with you our ongoing efforts in diversifying the structural repertoire of protein assemblies and developing strategies to dynamically control protein assembly state. First, I will describe our approach to the design of complex polyhedral geometries, in particular interlocked architectures of reduced porosity that can be controlled by pH changes. Then, I will present our module for interface-seeded assembly design in RFdiffusion, and how it can be used to generate symmetric or asymmetric oligomers mediated by simple, metal-dependent or small molecule-dependent protein-protein interfaces.
_________________
E 13
In-silico Design of Foldamer - Protein Interactions
Presenter: Hubert Klein (hubklph@cup.uni-muenchen.de)
Authors:
Hubert Klein
LMU - Ludwig-Maximilians-Universität München
Protein-protein interactions are common biomedical targets, as they are involved
in various diseases. We address the challenge of targeting flat protein-protein
interaction surfaces, which are inherently difficult to inhibit, by proposing the use
of non-natural aromatic delta peptides, namely foldamers. Foldamers give us
complete freedom of choice relative to amino acid sidechains, while retaining
design simplicity, as the foldamer secondary structure is robust to sidechain
identity changes. Here I will present a Rosetta-based pipeline to design foldamer-
binding proteins. Experimental validation will allow us to verify if the Rosetta
scoring function can properly rank interactions between proteins and foldamers.
In the future, this will help us establish a computational pipeline for the design of
foldamers to target specific PPIs.
_________________
E 14
Evaluation of cobalamin-binding chimeras designed by fold recombination
Presenter: Johanna-Sophie Koch (Johanna-Sophie.Koch@uni-bayreuth.de)
Authors:
Johanna-Sophie Koch¹; Prof. Dr. Sergio Romero Romero²; Dr. Saacnicteh Toledo-Patiño³; Alexander E. Braun¹; Prof. Dr. Birte Höcker¹
¹ University Bayreuth; ² Universidad Nacional Autónoma de México; ³ Okinawa Institute of Science and Technology
Modern-day proteins are very diverse. However, comprehensive analyses of sequences and structures reveal that our protein universe evolved through frequent re-usage of specific (sub)-domain-sized fragments, which can still be detected in contemporary proteins. Identification of such fragments inspired us to use them in a design approach by Chimeragenesis. The recombination of fragments from two (or more) proteins generates a chimera in which the fragment of one parent is replaced with one from another parent. Using this approach, a cobalamin-binding chimera (Cob-chimera) was designed by transferring a large part of the cobalamin-binding domain of methylmalonyl-CoA mutase (MCM) from Aeropyrum pernix into the uroporphyrinogen III synthase (U3S) from Homo sapiens giving rise to a stable chimera. Optimization led to further variants that improved the structure as well as the cobalamin binding. Here I will present in-depth studies of the chimeras’ abilities to bind cyano- or adenosylcobalamin. These new chimeric proteins on the one hand serve as a proof-of-principle that transfer of function between protein scaffolds is possible, and it further demonstrates chimeragenesis to be an innovative approach to construct proteins with novel properties.
_________________
E 15
Enriching Stabilizing Mutations through Analysis of Short Molecular Dynamics Simulations by BoostMut
Presenter: Kerlen Korbeld (k.t.korbeld@rug.nl)
Authors:
Kerlen Korbeld; Dr. Maximilian Fürst PhD
Rijksuniversiteit Groningen
Thermostability represents an important goal for protein engineers, e.g. to optimize enzymes for industrial biocatalysis. Despite much progress, the accuracy of thermostability predictors is still modest, even for state-of-the-art ML methods. To improve accuracy and reduce experiments, previously published in silico pipelines have combined the output of energy-based stability predictors with high-throughput molecular dynamics (MD) as a secondary filtering step. Although this method has been successful, a significant bottleneck to its application is the requirement of a visual inspection step that relies on the protein engineer’s chemical intuition and limits the reproducibility, speed, and throughput of the method.
Here we show the development and verification of BoostMut, a MDAnalysis-based command-line tool aiming to automate formalized and integrated checks previously achieved through inspection, including hydrogen bonds, solvent accessible surface, and flexibility. We show that BoostMut outcompetes human mutant filtering, and when we experimentally verified highly ranked mutations previously rejected, we achieve high success rates. As the BoostMut outputs provide a standardized dataset for each mutation, we also demonstrate its use as input for machine learning methods by training six models on the generated output, among which a random forest estimator showed the highest improvement in the prediction of stabilizing mutations.
_________________
E 16
Computational engineering of a metagenome-derived polyester hydrolase for efficient PET depolymerization
Presenter: Dr. Georg Künze (georg.kuenze@uni-leipzig.de)
Authors:
Dr. Georg Künze; Dr. Paula Blazquez-Sanchez; Jonas Gunkel; Dr. Abibe Useini; Dr. Alexander Zlobin; Dr. Christian Sonnendecker
Universität Leipzig
Current research focuses on the development of efficient polyester hydrolases for the recycling of polyester plastics such as Poly(ethylene terephthalate) (PET). The recently discovered Polyester Hydrolase Leipzig 7 (PHL7), isolated from a compost metagenome, completely degrades amorphous PET from post-consumer plastic waste in less than a day. However, its application at an industrial level has been hampered by its low stability and short lifetime at low salt concentrations and high temperatures, which are required for efficient PET hydrolysis. Here, we have applied a computer-aided design workflow to engineer PHL7 for improved stability and activity. Multiple mutations were predicted by Rosetta energy calculations and combined, increasing the thermal melting temperature to more than 95°C. Subsequent iterative mutagenesis around the PET binding pocket yielded a PHL7 variant with a >120-fold increased activity and 12.2°C higher melting temperature in 0.1 M buffer. X-ray structure and MD simulation analyses revealed the mechanisms underlying the enzyme’s elevated activity and stability. The new PHL7 variant is better than ICCG in terms of its PET hydrolyzing activity and comparable to the recently reported PETases LCC-A2 and TurboPETase at 65°C. Our results constitute a significant advancement for the engineering of industrially applicable PET hydrolases.
_________________
E 17
Developing Machine-Learning-Driven Sequence Design Strategies to Stabilize and Produce Challenging Enzymes
Presenter: Dr. Horst Lechner (horst.lechner@tugraz.at)
Authors:
Dr. Horst Lechner¹; Pedro D.G. Almeida Freitas¹; Ahmed Alagic¹; Dekel Cohen-Hoch¹; Markus Braun¹; Peter Macheroux¹; Gustav Oberdorfer
¹ Graz University of Technology
Many proteins of interest are marginally stable or challenging to express in heterologous hosts, limiting their use in biocatalysis and making them poor candidates for directed evolution. This project aims to successfully produce proteins of fungal and plant origin in heterologous expression systems. Instability often arises from intrinsic thermodynamic properties and evolutionary pressures specific to the organism’s environment. Existing computational tools like PROSS and FireProt2 can enhance protein stability and expression but rely on multiple sequence alignments, making them less effective for proteins with limited homologous sequences or from organisms that lack selective pressure to evolve stable proteins. This project seeks to develop a robust, generalizable method using machine-learning-based sequence design algorithms to improve protein production yields and stability. Advanced tools such as ProteinMPNN and language models like ESM-IF will be applied to optimize two challenging model enzymes: heme-containing unspecific peroxygenases and FAD-dependent berberine bridge enzymes, both of which are highly relevant in biocatalysis. This approach is anticipated to produce stable, highly expressible proteins that are independent of evolutionary constraints, offering valuable starting points for directed evolution campaigns and facilitating the study of hard-to-produce enzymes.
_________________
E 18
Functional Ligands: Protein design for electrochemical and structural control of ligand properties
Presenter: Bruce Lichtenstein (bruce.lichtenstein@port.ac.uk)
Authors:
Dr. Jessica Berrones; Alex Cahill; Matt Ellis; Dr. BRUCE LICHTENSTEIN¹
¹ UNIVERSITY OF PORTSMOUTH
Cofactors greatly extend the functional repertoire of proteins beyond the chemical and physical properties of natural amino acids. Despite their importance as critical components underlying almost all natural processes, very little is understood on how the protein environment acts to control their properties. To address this gap, we employ de novo design to systematically investigate how protein sequence and structure modulate the properties of bound cofactors, allowing us to isolate these effects from the evolutionary constraints of natural proteins. In this study, we are concurrently developing synthetic cofactors and de novo-designed scaffolds to enhance and regulate their activities. We present our latest findings on novel fluorogenic proteins and oxidoreductases, emphasizing recent advancements in designing cofactor-binding proteins for fully synthetic dyes, a new class of phenazines, and naturally inspired metallocorrins. This work builds on recent advances in ML-driven protein design, and we are testing the limits of these new algorithms to handle ligands well outside of natural systems.
_________________
E 19
Designing Protein Extensions: Key Puzzle Pieces for Artificial Molecular Motors
Presenter: Anna Zink (anna.zink@uni-bayreuth.de)
Authors:
Anna Zink; Dr. Vanessa Troßmann; Prof. Dr. Birte Höcker
Universität Bayreuth
The development of novel autonomous motor proteins relies on advanced protein design strategies. Linkers connecting two “feet” must enable precise and coordinated movement. Our study focuses on engineering extensions in the lactose repressor protein (LacI) as a step towards this goal.
Extensions were added to two insertion sites in LacI: (1) the C-terminus, and (2) a loop in the lower lobe of the PBP domain that shows significant dihedral angle differences between open and closed state. To design these extensions, we employed RFdiffusion and ProteinMPNN. Of six designs five retained the parental LacI sequence, while one was fully redesigned using ProteinMPNN. While all six proteins were successfully expressed, stability and solubility varied: two variants were found in inclusion bodies, one aggregated at low concentrations, and three appeared stable. Further characterisation of the four soluble variants revealed that three retained IPTG-binding and two DNA-binding capabilities. The single fully redesigned variant demonstrated excellent stability but lacked both functionalities.
Further efforts are directed towards resolving the structure of the functional variants and analysing the dynamics of the extensions using smFRET. This data will provide insights into how linker movement correlates with domain closure upon IPTG binding and lay the groundwork for connecting two “feet” of an artificial motor protein and mediate propagation of conformational changes.
_________________
E 20
Integrating geometric deep learning with explainable artificial intelligence to assist protein engineering
Presenter: Dr. David Medina-Ortiz PhD (dmedina@ipb-halle.de)
Authors:
Dr. David Medina-Ortiz PhD; Dr. Mehdi D. Davari PhD
Leibniz Institute of Plant Biochemistry (IPB)
Designing proteins with desirable properties has many applications in biotechnology. Traditional protein engineering approaches have benefited from advancements in artificial intelligence (AI), yet integrating AI into protein engineering introduces challenges in explainability and interpretability. Explainable Artificial Intelligence (XAI) addresses these challenges by providing insights into predictive model decision-making, identifying critical amino acid residues, uncovering physicochemical properties, and guiding protein design strategies transparently.
This work presents an XAI-based framework for protein design, integrating geometric deep learning, transformers, and XAI. Predictive models are developed using graph neural networks and embeddings from pre-trained protein language models. Graph-based methods, attention mechanisms, and feature engineering detect key residues and properties, enabling explanation of model predictions. These insights guide targeted modifications to enhance protein fitness by linking predictions to key residues and properties.
Validated on datasets like GB1 and TrpB, the framework identifies critical residues and properties that can improve protein fitness. The integration of generative strategies based on diffusion models is also being explored for E(3)-equivariant protein generation. This framework accelerates the design process and advances AI-driven protein engineering by enhancing interpretability, reliability, and predictive accuracy.
_________________
E 21
Engineering Cell Type Specific AAVs by Computational Protein Design
Presenter: Lennart Nickel (lennart.nickel@epfl.ch)
Authors:
Lennart Nickel; Christian Schellhaas; Martin Pacesa; Bernard Schneider; Bruno Correia
École Polytechnique Fédérale de Lausanne (EPFL)
Adeno-Associated Viruses (AAVs) are the most commonly used vectors in gene therapy, but their broad tropism can lead to liver toxicity and off-target effects. To address this, we present an AAV retargeting platform that leverages BindCraft, a novel computational pipeline for designing de novo binding proteins to functionalize the AAV capsid. By enforcing a minimal distance between the termini of the designed binder, BindCraft can be tuned to facilitate the integration into the capsid loops. BindCraft was benchmarked against several target proteins, achieving experimental success with just 10-20 designs per target.
As proof-of-concept, we engineered the AAV6 capsid by mutating glycan-interacting residues to create a knockout (KO) vector. We then inserted PD-L1-binding miniproteins into the KO vector, successfully producing retargeted chimeric AAVs in HEK293 cells. Mass spectrometry confirmed the successful incorporation of the miniprotein binder into the capsid. In vitro assays demonstrated selective transduction of PD-L1-expressing cells. This retargeting platform can be extended to other receptors, offering a streamlined approach for developing targeted gene therapies.
_________________
E 22
Accurate binder design using BindCraft
Presenter: Dr. Martin Pacesa PhD (martin.pacesa@epfl.ch)
Authors:
Dr. Martin Pacesa PhD
EPFL, École Polytechnique Fédérale de Lausanne
Protein–protein interactions (PPIs) are at the core of all key biological processes. However, the complexity of the structural features that determine PPIs makes their design challenging. We present BindCraft, an open-source and automated pipeline for de novo protein binder design with experimental success rates of 10-100%. BindCraft can generate binders with nanomolar affinity without the need for high-throughput screening or experimental optimization, even in the absence of known binding sites. We demonstrate binder designs against a diverse set of challenging targets, including cell-surface receptors, common allergens, de novo designed proteins, and multi-domain nucleases, such as CRISPR-Cas9. We showcase the functional and therapeutic potential of designed binders by reducing IgE binding to birch allergen in patient-derived samples, modulating Cas9 gene editing activity, and reducing the cytotoxicity of a foodborne bacterial enterotoxin. Lastly, we utilize cell surface receptor-specific binders to redirect AAV capsids for targeted gene delivery. This work represents a significant advancement towards a “one design-one binder” approach in computational design, with immense potential in therapeutics, diagnostics, and biotechnology.
_________________
E 23
Exploring enzymatic active sites using 3D point clouds representing physico-chemical properties of protein cavities
Presenter: Katharina Rauchenwald-Köchl (katharina.rauchenwald@innophore.com)
Authors:
Katharina Rauchenwald-Köchl; Chiara Gasbarri; Tobias Schopper; Marco Cespugli; Ursula Kahler; Bettina M. Nestl; Georg Steinkellner; Christian C. Gruber
Innophore GmbH
Highly efficient and selective enzymes are vital in prospective research areas within chemistry and biology. The discovery of new and improved enzymes represents a growing field with extensive applications across various sectors, including chemicals, pharmaceuticals, food production, biopolymers, and the synthesis of flavors and fragrances. The Catalophore™ platform synergizes bioinformatics methods with artificial intelligence to search structural enzyme and drug databases, identifying potential enzyme candidates suitable for industrial processes, biochemical reactions, and therapeutic applications across various domains.
The Catalophore™ technology focuses on analyzing the binding sites, referred to as active sites or cavities, within enzymes.These active sites are mapped using the patented 3D point-cloud technology encompassing up to 19 physico-chemical properties, such as electrostatics, hydrophobicity, accessibility, potential energies, hydrogen bonding potential, elasticity, etc.
Herein, we present how we systematically examine enzymatic active sites using our Catalophore™-driven deep learning approach, which serves to complement traditional methodologies, including structural and sequential comparisons. Our explorative designs have resulted in the identification of promising enzyme variants exhibiting significantly enhanced activities.
_________________
E 24
Two-state design enabled the reliable optimisation of dynamic small molecule binding proteins
Presenter: Marc Scherer (marc.scherer@uni-bayreuth.de)
Authors:
Marc Scherer¹; Prof. Dr. Sarel J. Fleishman²; Prof. Dr. Birte Höcker¹
¹ University of Bayreuth; ² Weizmann Institute of Science
Having control over specific responses to stimuli is pivotal in the optimization of protein-based nanoswitches. However, a reliable pipeline for the computational design of dynamic proteins has not been described yet. Here we demonstrate how evolution-based atomistic design affects the conformational dynamics of periplasmic binding proteins (PBPs) upon interaction with target molecules. We suspect that designing thermostability of dynamic proteins based on a single structure can alter the conformational equilibrium and hence binding functionality. In consequence, an advanced strategy is suggested that employs two-state design and interface constraints to improve its reliability. Based on the experimental characterisation of 16 PBP designs, we validate this hypothesis and can show that although all designs have higher melting points, some single state designs have decreased affinity to their cognate ligands. This study gains insights into the mechanisms governing protein conformational rearrangements and offers a strategy for the one-shot design of thermostable and responsive small molecule binders.
_________________
E 25
From Genome Mining to Enzyme Engineering: A case study one the Phosphoramidon Biosynthetic Pathway
Presenter: Phillip Schlegel (phillip.schlegel@medizin.uni-leipzig.de)
Authors:
Phillip Schlegel; Matheus R. Belisário-Ferrari; Prof. Dr. Leonard Kaysser; Jun.-Prof. Dr. Clara T. Schoeder
Leipzig University, Institute for Drug Discovery
Phosphoramidon, a potent metalloprotease inhibitor, holds substantial therapeutic and research potential due to its unique structure, featuring a dipeptide backbone linked to a 6-deoxysugar via a phosphoramidate bridge. While the general biosynthetic pathway of phosphoramidon has been proposed, the precise enzymatic reactions have not been fully characterized. Genome mining efforts previously identified key enzymes involved in its biosynthesis, providing a foundation for further investigation. Building on these findings, this study reconstitutes the remaining biosynthetic steps in vitro, offering a detailed understanding of the pathway. A central focus is the ATP-grasp enzyme TalD, which catalyzes the formation of the dipeptide backbone. TalD’s substrate selectivity and thermal stability were investigated, revealing its potential as a versatile biocatalyst for engineering bioactive small molecules. Additionally, targeted mutations, designed using computational tools, were introduced to refine TalD’s biocatalytic properties. This work confirms the catalytic roles of the identified enzymes, laying the groundwork for future enzyme engineering initiatives and demonstrating the power of combining genome mining, synthetic biology, and computational protein design in the development of bioactive small molecule.
_________________
E 26
Analysis and design of protein fold switches
Presenter: Lisa Schmidt (lisa.schmidt@mpinat.mpg.de)
Authors:
Lisa Schmidt; Bert L. de Groot
Max Plank Institute for Multidisciplinary Sciences
Protein fold-switches are important in many biological processes and exhibit great structural diversity. This makes them perfect for protein design studies with the aim of creating programmable proteins with new functions. Although protein design has made remarkable progress in recent years, design of fold-switching proteins de novo is still a challenging task.
We use MD simulations and existing ML tools to analyze different switches. To obtain a deeper understanding of the requirements of fold switching, we combine free energy calculations with dynamical, structural, and sequence analysis of existing switches. Furthermore, we use our methods to introduce controls to existing switches and design novel switch systems.
_________________
E 27
Protein Design Yielding Complex Functions in Synthetic Cells
Presenter: Andrey Sysoev (sysoev@biochem.mpg.de)
Authors:
Andrey Sysoev; Béla Frohn; Dr. Kareem Al Nahas; Prof. Dr. Lukas Milles; Prof. Dr. Petra Schwille
Max Planck Institute for Biochemistry
Protein design offers efficient tools for creating simple molecular functions, and the field is challenged to advance towards complexity. One way is to design simple functions and then integrate them with other components. Concurrently, bottom-up synthetic biology aims to engineer biological functional modules, such as metabolism, signaling or division, using a minimal set of purified molecules. Therefore, bottom-up synthetic biology provides an ideal environment for realizing the potential of protein design to create complex functions. One of the most important functional modules is cell division, which is currently the least developed module. In lipid vesicles, the bacterial tubulin homolog FtsZ was shown to polymerize into a ring-like structure along the inner membrane and to bend the membrane inward, thereby pinching the vesicle roughly in the middle. However, full fission has not been achieved yet, and we hypothesize that it might be crucial to induce FtsZ disassembly after ring formation. To this end, we use RFdiffusion to design binders to the FtsZ, with the aim of inducing depolymerization. We develop a high-throughput experimental screening pipeline that enables us to identify, from the set of 95 designed proteins, those that confidently bind to FtsZ monomers, alter FtsZ polymerization dynamics in bulk, and depolymerize assembled FtsZ rings in synthetic cells. This work demonstrates that protein design can already be used to engineer complex biological functions.
_________________
E 28
Flexibility-conditioned protein structure design
Presenter: Vsevolod Viliuga (vsevolod.viliuga@gmail.com)
Authors:
Vsevolod Viliuga¹; Leif Seute²; Nicolas Wolf²; Simon Wagner³; Prof. Arne Elofsson¹; Jun. Prof. Jan Stühmer²; Prof. Frauke Gräter⁴
¹ SciLifeLab; ² HITS gGmbH; ³ IWR, Heidelberg University; ⁴ MPIP - Mainz
Recent advances in geometric deep learning and generative modeling have enabled the design of novel proteins with a wide range of desired properties. However, current state-of-the-art approaches are typically restricted to generating proteins with only static target properties, such as motifs and symmetries, which are often incorporated in over-stabilized, rigid protein structures. In this work, we take a first step towards overcoming this limitation by proposing a framework to condition structure generation on local flexibility, which is crucial for key functionalities such as catalysis or chemical reactivity. We first introduce BackFlip, an equivariant graph neural network for predicting per-residue flexibility from an input backbone structure. With the help of BackFlip, we develop GAFL-Flex, an SE(3) equivariant flow matching model that solves the inverse problem of generating backbones that display a target flexibility profile. In our experiments, we demonstrate that GAFL-Flex is able to generate diverse and novel protein backbones with the desired local flexibility, verified by Molecular Dynamics (MD) simulations.
_________________
E 29
A multifaceted approach for efficient protein translocation and direct purification of in vitro synthesized protein
Presenter: Leo von Bank (Bank@mpi-marburg.mpg.de)
Authors:
Leo von Bank; Markus Meier; Dr. Scott Scholz; Prof. Dr. Tobias J. Erb
MPI for Terrestrial Microbiology, Marburg
The channel protein SecYEG & the motor SecA drive protein translocation ubiquitous throughout life. Translocated proteins only require an N-terminal Signal Peptide for recognition. Therefore, SecYEG is an interesting target for biotechnological applications and is exploited for the secretion of proteins. However, these studies were based on time consuming readouts.
We can test the translocation activity in 6 µl under 5 h. For that, the post-translational translocation pathway is reconstituted in a cell-free expression system and measured in real time by luminescence. Each component is defined and can be easily exchanged. This makes the assay highly amenable for high-throughput screening and AI data acquisition.
To enhance translocation activity, we engineer all parts of the translocation machinery, (1) the lipid environment including synthetic lipids, (2) the SecYEG channel, (3) SecA as motor, (4) the signal peptide, (5) and the translocated substrate.
With our work we showcase a multifaceted approach that improves in vitro translocation of cell-free expressed protein. Finally, we will use our findings for direct protein purification to demonstrate real life application.
For future work the SecYEG-A t machinery can be engineered by machine learning algorithms. Additionally, fitness landscapes could be generated to provide further insides into an ubiquitous part of life.
_________________
E 30
Generating Highly Designable Proteins with Geometric Algebra Flow Matching
Presenter: Simon Wagner (simon.wagner@iwr.uni-heidelberg.de)
Authors:
Simon Wagner; Leif Seute; Vsevolod Viliuga; Nicolas Wolf; Prof. Dr. Frauke Gräter; Jun.-Prof. Dr. Jan Stühmer
We introduce a generative model for protein backbone design utilizing geometric products and higher order message passing. In particular, we propose Clifford Frame Attention (CFA), an extension of the invariant point attention (IPA) architecture from AlphaFold2, in which the backbone residue frames and geometric features are represented in the projective geometric algebra. This enables to construct geometrically expressive messages between residues, including higher order terms, using the bilinear operations of the algebra. We evaluate our architecture by incorporating it into the framework of FrameFlow, a state-of-the-art flow matching model for protein backbone generation. The proposed model achieves high designability, diversity and novelty, while also sampling protein backbones that follow the statistical distribution of secondary structure elements found in naturally occurring proteins, a property so far only insufficiently achieved by many state-of-the-art generative models.
_________________
E 31
Computational design of sequence-specific peptide binders using AF2
Presenter: Jonas Wepfer (j.wepfer@bioc.uzh.ch)
Authors:
Jonas Wepfer; Gwendal Loarer; Prof. Dr. Amedeo Caflisch; Prof. Dr. Andreas Plückthun
Designed Armadillo Repeat Proteins (dArmRPs) offer considerable potential for the production of custom-designed binding proteins for linear epitopes targeting, applied to biologic research and biotherapeutics. This study introduces a novel computational pipeline integrating AlphaFold2 (AF2) with a genetic algorithm (GA) to selectively re-target dArmRPs against novel epitopes. We test the approach by selectively retargeting dArmRPs against the peptide of interest Nter-KAKIKIARQRGG-Cter. The GA efficiently samples the sequence space, enriching binders with the desired interaction conformation. The presence of persistent subclusters of sequences across generations indicates a high diversity of enriched sequences. This diversity was experimentally confirmed by comparing the evolved sequences with NGS data from a directed evolution experiment using yeast surface display. The analysis of the AF2 scoring revealed a higher sensitivity towards residues positioned proximal to the target binding interface. To enhance reliability, a phylogenetic close-neighbor analysis method, effectively discriminating against false positives, was developed. Finally, we experimentally characterized the designed binders, with 20% showing a dissociation constants below 10 nM. One of the designs displaying high specificity against similar peptide sequences. Overall, this study showcases the use of AF2 combined with a GA as a reliable method for the design of highly affine and sequence-selective dArmRPs.
_________________
E 32
Computational design of biosensors and point of care diagnostics for emerging synthetic opioids
Presenter: Prof. Timothy Whitehead PhD (timothy.whitehead@colorado.edu)
Authors:
Prof. Timothy Whitehead PhD
University of Colorado, Boulder
Synthetic opioids known as nitazenes - defined by a shared 2-benzyl benzimidazole pharmacore - may be significantly more potent than fentanyl and have recently been detected internationally in illicit drugs. New ways of testing the range of nitazenes, and metabolic byproducts of nitazenes, are urgently needed. We developed a computational protocol to redesign the plant abscisic acid receptor PYR1 to recognize diverse nitazenes. The best sensors have low nanomolar limits of detection in vitro against nitazene and menitazene. Deep mutational scanning yielded a sensor able to recognize a range of clinically relevant nitazenes in complex biological matrices with limited cross-specificity against unrelated opioids. Application of protein design tools on privileged receptors like PYR1 may yield general sensors for a wide range of applications in vitro and in vivo.
_________________
E 33
Rational engineering of allosteric protein switches by in silico prediction of domain insertion sites
Presenter: Benedict Wolf (benedict.wolf@uni-heidelberg.de)
Authors:
Benedict Wolf; Pegi Shehu; Luca Brenker; Anna von Bachmann; Ann-Sophie Kroell; Nicholas Southern; Stefan Holderbach; Joshua Eigenmann; Sabine Aschenbrenner; Dr. Jan Mathony; Prof. Dr. Dominik Niopek
Uni Heidelberg
Domain insertion engineering is a powerful approach to juxtapose otherwise separate biological functions, resulting in proteins with new-to-nature activities. A prominent example are switchable protein variants, created by receptor domain insertion into effector proteins. Identifying suitable, allosteric sites for domain insertion, however, typically requires extensive screening and optimization.
We present ProDomino, a novel machine learning pipeline to rationalize domain recombination, trained on a semi-synthetic protein sequence dataset derived from naturally occurring intradomain insertion events. ProDomino robustly identifies domain insertion sites in proteins of biotechnological relevance, which we experimentally validated in E. coli and human cells. Finally, we employed light- and chemically regulated receptor domains as inserts and demonstrate the rapid, model-guided creation of potent, single-component opto- and chemogenetic protein switches. These include novel CRISPR-Cas9 and -Cas12a variants for inducible genome engineering in human cells. Our work enables one-shot domain insertion engineering and substantially accelerates the design of customized allosteric proteins.
_________________
E 34
Damietta protein design toolkit
Presenter: Tobias Wörtwein (tobias.woertwein@tuebingen.mpg.de)
Authors:
Tobias Wörtwein¹; Mohammad ElGamacy¹; Kateryna Maksymenko²; Iwan Grin
¹ University Hospital Tübingen; ² Max plank institut for Biology
We are developing general-purpose protein design software suite as well as the graphical web-interface for broader user access. Damietta is a physics-based protein design tool which uses tensorized energy calculations and molecular dynamics-derived rotamer library. Here, I present key parts of the workflow, different protocols in the software suite, and the functionality available through the toolkit webserver (available at: https://damietta.de). I also present our new recent developments within the damietta framework that enable molecular docking, as well as the design of heterochiral proteins.
_________________
E 35
AI-Driven Design of Chimeric Antigen Receptor Binders
Presenter: Christina Wüst; Johannes Klier (christina.wuest@medizin.uni-leipzig.de)
Authors:
Christina Wüst¹; Johannes Klier¹; Florian Große²; Dr. Kristin Reiche²; Jun.-Prof. Dr. Clara T. Schoeder¹
¹ Leipzig University; ² Fraunhofer Institute for Cell Therapy and Immunology IZI
Chimeric antigen receptor (CAR) T cell therapy has transformed cancer treatment by enabling precise tumor targeting. However, antigen heterogeneity and isoform variation pose major challenges, often leading to resistance or reduced efficacy of CAR T cell-based therapies. To address this, we present an AI-driven designing pipeline for de novo binders against clinically relevant tumor isoforms.
Our pipeline characterizes targets based on clinical data on CAR T cell treatment responses, combined with spatial transcriptomics to identify isoform-specific epitopes. By considering isoforms, we can either design binders that target tumor-associated isoforms to
enhance specificity or focus on isoform-independent epitopes to improve the robustness of CAR T
cell therapies. AlphaFold has major limitations when predicting isoforms due to structure memory. Here, we employ a score to differentiate valid models from AlphaFold.
De novo binder constructs are generated using RFdiffusion, optimized with ProteinMPNN, and structurally validated via AlphaFold-based interaction prediction. Candidate binders are filtered based on predicted stability, affinity, and specificity.
This work highlights the potential of AI-driven protein design to overcome current limitations in immunotherapy.
_________________
E 36
AI-based redesign improves catalytic efficiency and enantioselectivity of an artificial photoenzyme
Presenter: Prof. Dr. Cathleen Zeymer (cathleen.zeymer@tum.de)
Authors:
Florian Leiss-Maier; Paula Wagner Egea; Joshua Behringer; Ghulam Mustafa; Andreas Sebastian Klein; Prof. Dr. Cathleen Zeymer
TU München
Cerium photoredox catalysis is a powerful method to activate organic molecules under mild conditions. However, it remains a major challenge to achieve stereocontrol in these light-driven radical reactions. We thus developed a cerium-dependent photoenzyme enabling this chemistry in the chiral environment of a de novo protein. Our work is based on a de novo TIM barrel scaffold designed previously in a physics-based approach [1,2]. We equipped the protein with a high-affinity metal binding site for lanthanide ions [2] and demonstrated its photocatalytic potential. Upon visible-light irradiation, the cerium-bound enzyme enables the radical C–C bond cleavage of 1,2-diols in aqueous solution [3]. To optimize the initially low activity and enantioselectivity, we redesigned the scaffold computationally. We decreased the cavity size between the two independently folded domains and utilized the deep-learning tool “ProteinMPNN” [4] to redesign the sequences. Selected redesigns were characterized experimentally and showed significantly improved kcat/KM and enantiomeric excess in kinetic resolutions of diols.
References
[1] Huang et al. (2016) Nat. Chem. Biol. 12, 29-34
[2] Caldwell et al. (2020) Proc. Natl. Acad. Sci. U.S.A. 117, 30362-30369
[3] Klein et al. (2024) J. Am. Chem. Soc. 146, 25976-25985
[4] Dauparas et al. (2022) Science 378, 49-56
_________________
E 37
Reprogramming self-inserting nanopores for differential transport across synthetic membranes
Presenter: Anastasia Götz (elisabeth.bobkova@mpi-marburg.mpg.de)
Authors:
Elisabeth Bobkova; Anastasia Götz; Luis Felipe Gutierrez-Mondragon; Dr. Scott Scholz; Prof. Dr. Tobi Erb
MPI for Terrestrial Microbiology, Marburg
Engineering membrane proteins with specific functions is a significant challenge in synthetic biology, hindered by difficult characterization and the lack of scalable, high-throughput methods. Self-inserting nanopores typically lack defined transport functions, while functional pores with selective transport often fail to self-insert.
To address this, we engineered the self-inserting nanopore alpha-Hemolysin, to enable selective transport through linker functionalization. By employing controllable modifications with Methanethiosulfonate (MTS) reagents and peptides, we developed an efficient method to functionalize the pores at different sites, both pre- and post-membrane insertion. We further adapted plate reader compatible assays to screen and characterize transport changes of small molecules and peptides.
The presented pipeline allows functionalization and testing of hundreds of pore variants, allowing for AI-ready data generation that can be leveraged to further optimize and customize transport properties. These advances pave the way for spatially controlled bioreactions, synthetic cell systems, and functionalized biomaterials.
_________________
M 01
Predicting interactions of proteins with metals using cofolding methods and AllMetal3D
Presenter: Dr. Simon Dürr (simon.duerr@epfl.ch)
Authors:
Dr. Simon Dürr; Prof. Dr. Ursula Rothlisberger
Ecole Polytechnique Fédérale Lausanne
Nature uses a variety of metal ions as cofactors for specific tasks such as catalysis or structural stability. The three main classes of metals in biological systems are alkali ions (e.g. sodium and potassium), alkaline earth ions (e.g. magnesium and calcium) as well as transition metal ions (e.g. zinc or iron). In this contribution, we introduce AllMetal3D to predict not only the location of metal ions but also the selectivity of a given protein for a specific metal ion. AllMetal3D predicts all important biological metals with high spatial precision and can be used as part of experimental protein structure determination at the refinement stage. We showcase important design decisions for classification problems in structural biology that if ignored can lead to misleading performance. We also contrast the performance of cofolding methods such as AlphaFold3 and RoseTTAfold-AllAtom with AllMetal3D and highlight further avenues of improvement for these models.
_________________
M 02
Predicting Molecular Hotspots for Efficient Drug Discovery using Deep Learning
Presenter: Inken Fender (inkne.fender@unibe.ch)
Authors:
Inken Fender; Prof. Thomas Lemmin
University of Bern
Protein-ligand interactions play a central role in many biological processes ranging from cell signaling to disease treatment. However, traditional drug discovery struggles with the immense number of potential drug molecules and the limited availability of detailed protein-ligand complex data. This bottleneck hinders the development of highly selective drugs with strong binding affinity to their target proteins. Recent research has opened an exciting new avenue by demonstrating the potential to predict the most probable orientations and positions of chemical groups solely from the local backbone conformation and the identity of the interacting amino acid. This eliminates the need for scarce protein-ligand complex data, offering a significant advantage in drug design.
Our research builds upon this promising avenue and takes a significant step forward by extending the concept to the broader protein micro-environment. We propose formulating the identification of functional hotspots within these micro-environments as a semantic segmentation task. Our method harnesses a convolutional neural network (CNN) trained on a meticulously curated database, encompassing a diverse array of protein micro-environments surrounding various functional groups. To assess the efficacy of our approach, we evaluated the predicted hotspots based on their pharmacological relevance and compared them to existing protein-ligand complexes.
_________________
M 03
Synthetic MSAs identify protein conformations
Presenter: Jannik Adrian Gut (jannik.gut@unibe.ch)
Authors:
Jannik Adrian Gut; Noah Kleinschmidt; Thomas Lemmin
Universität Bern
Protein folding is a crucial task in structural biology and involves predicting the 3D structure of a protein from its amino acid sequence. A protein's structure provides critical insights into its functions and interactions with other biomolecules. Recently, AlphaFold has revolutionized the field by enabling the accurate prediction of high-quality protein structures. A significant challenge that still remains is accurately predicting alternative conformations that a protein can adopt under different conditions.
Previous research has demonstrated that AlphaFold can predict alternative conformations by manipulating its input. AlphaFold relies on two types of input: multiple sequence alignments (MSAs) and structural templates obtained from vast databases. Among these, MSAs have a more significant influence. However, the manipulation of the input often fails when tasked with predicting more complex or challenging conformations.
To address this, we leverage inverse folding models to generate protein sequences that form one MSA for a given structure. These synthetic MSAs, when fed into AlphaFold, significantly outperform both the standard AlphaFold pipeline and conformation-optimized methods. Our approach demonstrates broader applicability, extendability to other protein folding models and independence of close homologs. This work presents a novel paradigm for integrating template information into AlphaFold and offers a new strategy for sampling alternative protein conformations.
_________________
M 04
Learning conformational ensembles of proteins based on backbone geometry
Presenter: Leif Seute (leif.seute@h-its.org)
Authors:
Nicolas Wolf¹; Leif Seute²; Vsevolod Viliuga³; Simon Wagner⁴; Jan Stühmer²; Frauke Gräter¹
¹ Max Planck for Polymer Research; ² Heidelberg Institute for Theoretical Studies (HITS gGmbH); ³ SciLifeLab; ⁴ IWR, Heidelberg University
Deep generative models have recently been proposed for sampling protein conformations from the Boltzmann distribution, as an alternative to often prohibitively expensive Molecular Dynamics simulations. However, current state-of-the-art approaches rely on fine-tuning pre-trained folding models and evolutionary sequence information, limiting their applicability and efficiency, and introducing potential biases. In this work, we propose a flow matching model for sampling protein conformations based solely on backbone geometry. We introduce a geometric encoding of the backbone equilibrium structure as input and propose to condition not only the flow but also the prior distribution on the respective equilibrium structure, eliminating the need for evolutionary information. The resulting model is orders of magnitudes faster than current state-of-the-art approaches at comparable accuracy and can be trained from scratch in a few GPU days. In our experiments, we demonstrate that the proposed model achieves competitive performance with reduced inference time, across not only an established benchmark of naturally occurring proteins but also de novo proteins, for which evolutionary information is scarce.
_________________
M 05
Towards a comprehensive view of the pocketome universe – biological implications and algorithmic challenges
Presenter: Hanne Zillmer (zillmer@mpimp-golm.mpg.de)
Authors:
Hanne Zillmer; Dirk Walther
Max Planck Institute of Molecular Plant Physiology
With the availability of reliably predicted 3D-structures for essentially all known proteins, characterizing the entirety of protein - small-molecule interaction sites (binding pockets) has become a possibility. The aim of this study was to identify and analyze all compound-binding sites, i.e. the pocketomes, of eleven different species’ from different kingdoms of life to discern evolutionary trends as well as to arrive at a global cross-species view of the pocketome universe. All protein structures available in the AlphaFold database for each species were subjected to computational binding site predictions. The resulting set of potential binding sites was inspected for overlaps with known pockets and annotated with regard to the protein domains. 2D-projections of all pockets embedded in a 128-dimensional feature space and characterizing all pockets with regard to selected physicochemical properties, yielded informative, global pocketome maps that reveal differentiating features between pockets. By clustering all pockets within species, our study revealed a sub-linear scaling law of the number of unique binding sites relative to the number of unique protein structures per species. Thus, larger proteomes harbor less than proportionally more different binding sites than species with smaller proteomes. We discuss the significance of this finding as well as identify critical and unmet algorithmic challenges.
_________________
M 06
Structural Insights into an Anaerobic CO₂ Fixation Complex
Presenter: Dr. Max Dongsheng Yin (mayin@biophys.mpg.de)
Authors:
Dr. Max Dongsheng Yin
Contribution withdrawn
_________________
O 01
Enforced phosphatase recruitment as a novel strategy in the treatment of acute myeloid leukemia
Presenter: Khadija Aichane-Simon (Aichane-Simon.Khadija@mh-hannover.de)
Authors:
Khadija Aichane-Simon
Hannover Medical School
Acute myeloid leukemia (AML) is a common form of blood cancer with a high mortality rate. The receptor tyrosine kinase (RTK) FMS-related tyrosine kinase 3 (FLT3) is one of the most frequently mutated genes in AML, accounting for 15–35% of cases. Although small molecule-based tyrosine kinase inhibitors, like Gilteritinib, have been developed for the treatment of AML, a lack of specificity, off-target effects and the development of drug resistance are common issues. A new strategy to inhibit RTK signaling is by phosphatase recruitment, e.g. through the recruitment of the receptor-like tyrosine phosphatase CD45. The adenoviral protein E3/49K contains two domains that bind CD45, thereby inhibiting its phosphatase activity by dimerization and preventing immune cell activation. Thus, a fusion protein of FLT3 ligand (FLT3L) and the CD45-binding domain R2 of E3/49K could mediate enforced phosphatase recruitment of CD45 to FLT3. Our aim was to test the applicability of a 49K-FLT3L fusion protein as a potential treatment for AML by inhibiting FLT3 signaling and cell proliferation in the AML cell line MV4-11. Indeed, binding of 49K-FLT3L to CD45 and FLT3 on MV4-11 cells resulted in decreased phosphorylation of FLT3 and its downstream targets ERK and AKT. In addition, cell proliferation was reduced and apoptosis was induced in these cells. Thus, the enforced phosphatase recruitment may be a promising strategy in the treatment of AML, especially in combination with already existing drugs.
_________________
O 02
Design and optimization of flavoproteins for hyperpolarized EPR methods
Presenter: Varvara Apet MSc. (varvara.apet@uni-leipzig.de)
Authors:
Varvara Apet MSc.; Sebastian Krebs; Felipe Engelberger; Prof. Dr. Jens Meiler; Dr. Matthias Elgeti; Prof. Dr. Irene Coin
Leipzig University
Electron Paramagnetic Resonance (EPR) and Nuclear Magnetic Resonance (NMR) are crucial techniques in structural biology, providing excellent spatial resolution and the capability to track fast molecular processes. Nevertheless, the sensitivity of these techniques is restricted by low polarization, which is a consequence of an unfavourable Boltzmann distribution. To overcome this shortcoming, we aim to develop a biological, hypersensitive spin tag for hyperpolarized EPR spectroscopy. Our initial focus involves flavoprotein sensors such as Light-Oxygen-Voltage (LOV) domains, Blue Light-Using Flavin (BLUF) domains, and cryptochromes, which naturally generate spin-correlated radical pairs (SCPR) upon illumination between flavin and aromatic amino acids. These SCPR proteins will be used as fusion tags for G protein-coupled receptors (GPCRs) in double electron-electron resonance (DEER) experiments. The ultimate goal of this project is to design a de novo protein tag, termed "maquette", that maintains the desired functional properties while featuring simplified structural characteristics. Beyond structural studies, this tag will facilitate investigations into electron transfer and hyperpolarization mechanisms across different protein structures, including α-helices and β-sheets. The hyperpolarized electron-spin system will be a valuable tool for in-cell EPR experiments on samples at very low concentrations.
_________________
O 03
Predicting structural features of suppressor tRNAs using MD simulations
Presenter: Stine Behrmann (stine.behrmann@uni-hamburg.de)
Authors:
Stine Behrmann; Daniel Köster; Christine Polte; Yanqi Jiao; Dr. Suki Albers; Prof. Dr. Zoya Ignatova
The design of tRNAs to suppress premature termination codons (PTC) - the underlying cause for approximately 11% of the genetic diseases - remains a big challenge. Our previous work highlights the therapeutic potential of suppressor tRNAs engineered to efficiently decode PTCs and re-establishe protein expression and function in the patient-derived cells and thus, would alleviate the disease phenotype. However, the design process of suppressor tRNAs remains a challenge. The idiosyncratic connections between the nucleotides in tRNAs makes rational predictions of sequence changes to improve activity difficult. Thus, we employ MD simulation and compare the behavior of wildtype and suppressor tRNAs. We analyze the simulation data regarding the stability and flexibility of the tRNAs using RMSD, RMSF and hydrogen bonds. Comparison between wildtype and suppressor tRNAs enable identifying trends which correlate with the efficiency of the suppressor tRNAs. we combine these in silico data with experimental measurements of the suppressor’s readthrough efficiencies. Collectively, these data are used in a machine learning algorithm to recognize patterns in the tRNAs, that will facilitate the design of suppressor tRNAs. This will advance the development of highly efficient suppressor tRNAs, thereby providing therapeutic options for patients with PTC-associated diseases.
_________________
O 04
Bicelles assembled with the G protein-coupled receptor rhodopsin as model lipid-protein system for interaction studies
Presenter: Patryk Bielski (patryk.bielski@uni-oldenburg.de)
Authors:
Patryk Bielski¹; Justyna Bożek; Dr. Izabella Brand; Prof. Dr. Karl-Wilhelm Koch
¹ Carl von Ossietzky Universität Oldenburg
Transmembrane proteins (TMPs) are found in almost all organisms, and they play critical roles for cell metabolism, inter- and intra-cellular communication, control of the electrical and ion balances, maintenance of the cell structure integrity and cell adhesion. Understanding their structure and behaviour in their natural environment is very important to investigate membrane proteins biological functions. Purification of TMPs requires removal of the native lipid matrix bearing the risk of losing the native structure and function of the protein. Incorporation of TMPs in bicelles – two-dimensional lipid discs, composed of long- and short-chain lipids provide a native-like lipid environment for TMPs. We investigated the prototypical G protein coupled receptor (GPCR) rhodopsin to address the following research topics: incorporation of rhodopsin into bicelles, biophysical characterization of bicelles in the absence and presence of rhodopsin, structural information obtained by infrared spectroscopy. Since bicelles are able to spread onto solid supports to form lipid bilayers we immobilized bicelles for protein-lipid and protein-protein interaction analysis using surface plasmon resonance.
_________________
O 05
Multimerization of Grb2 revealed by the Grb2-Gab1(497-528) complex structure
Presenter: Dr. Constanze Breithaupt (constanze.breithaupt@biochemtech.uni-halle.de)
Authors:
Dr. Constanze Breithaupt¹; Dr. Tobias Gruber; Dr. Katharina Mandel; Dr. Marc Lewitzky; Dr. Annette Meister; Prof. Jochen Balbach; Prof. Stephan Feller; Prof. Milton T. Stubbs
¹ Martin- Luther- Universitaet Halle- Wittenberg
Growth factor receptor bound protein 2 (Grb2) plays an essential role in signal transduction by binding to activated receptor tyrosine kinases through its SH2 domain and to downstream effectors, such as the guanine nucleotide exchange factor SOS or the multi-site docking protein Grb2-associated binder-1 (Gab1), via its N- and C-terminal SH3 domains (nSH3, cSH3). Here we present the first experimental structure of full length Grb2 bound to an effector peptide. The crystal structure of Grb2 in complex with the bidentate nSH3-cSH3-binding peptide Gab1497-528 provides molecular insight into the recognition of SH3-binding effector proteins by Grb2 and reveals the assembly of a two-dimensional meshwork, consisting of multimeric filament-like Grb2 chains linked to each other by the bivalent bound Gab1497-528 peptide. Dominant contacts between Grb2 molecules in the structure of the multimer are provided by an intermolecular SH2/cSH3 domain interface, leaving kinase and effector binding sites freely accessible. We further show that Grb2 is able to form phase-separated condensates in solution, a process that depends on the presence of an intact SH2/cSH3 interface and that is fostered by addition of Gab1497-528, in line with the multimeric assembly seen in the crystal structure.
_________________
O 06
Validation and characterization of a fourth preQ₁ riboswitch class
Presenter: Jan A. Dewald (jan.dewald@uni-leipzig.de)
Authors:
Jan A. Dewald¹; Thilo Schmid²; Paul M. Tarlatt²; Felix Wenzek³; Sabine Müller³; Zasha Weinberg²; Christina E. Weinberg¹
¹ Martin-Luther Universität Halle-Wittenberg; ² Universität Leipzig; ³ Universität Greifswald, Institut für Biochemie
Riboswitches are structured RNAs, mostly located in 5ˈ untranslated regions of bacterial messenger RNAs. They are able to regulate gene expression upon specific binding to a ligand. To date, over 55 naturally occurring riboswitch classes are known with some being extensively used in various applications in synthetic biology.
We characterized a novel riboswitch motif identified in environmental samples by comparative sequence analyses. All representatives are associated with the queT gene encoding a transport protein previously shown to be involved in preQ1-transport. PreQ1 (7-aminomethyl-7-deazaguanine) is a precursor of the highly modified guanine nucleotide queousine that functions as an essential tRNA modification in bacteria and eukaryotes. We verified the bioinformatically predicted structure and ligand binding ability of the riboswitch by in-line probing. Furthermore, its ability to regulate gene expression in vivo was proven using lacZ reporter gene assays. Attempts are currently made to solve the 3D crystal structure of this novel riboswitch class to decipher how it binds its ligand hopefully providing new interesting insights into RNA folding.
With this work, we present a fourth preQ1 riboswitch class, further extending the existing spectrum of naturally occurring riboswitch classes, expanding the toolbox for synthetic biology applications and potentially elucidating new RNA folds.
_________________
O 07
A core network in SARS-CoV-2 nucleocapsid NTD drives domain stability and RNA recognition.
Presenter: Karthikeyan Dhamotharan (dhamotharan@bio.uni-frankfurt.de)
Authors:
Karthikeyan Dhamotharan¹; Dr. Sophie M. Korn²; Dr. Anna Wacker¹; Matthias A. Becker¹; Dr. Sebastian Günther³; Dr. Cy M. Jeffries⁴; Prof. Dr. Harald Schwalbe¹; Prof. Dr. Andreas Schlundt⁵
¹ Center for Biomolecular Magnetic Resonance (BMRZ), Goethe University; ² Department of Biochemistry and Molecular Biophysics, Columbia University; ³ Center for Free-Electron Laser Science CFEL, Deutsches Elektronen-Synchrotron DESY; ⁴ European Molecular
The nucleocapsid (N) is one of the most abundant proteins during SARS-CoV-2 infection. N plays multifaceted roles in recognizing and binding functional RNA elements involved in replication, transcription, and packaging. N’s RNA-binding specificity is primarily attributed to its N-terminal RNA-binding domain (NTD). The 3D structure of NTD features β-strand elements connected by flexible loops and a β-hairpin, resembling a hand with fingers. These flexible “fingers” allow NTD to engage effectively with target RNAs. However, the lack of atomic structures of the NTD in complex with biologically relevant RNA limits our understanding of this intricate mechanism. Moreover, viral evolution has introduced mutations within the NTD in several variant lineages. As the functions of the N protein are closely tied to RNA-binding, these mutations in the NTD carry essential implications. Our study explored the effects of natural and strategically introduced mutations on the structural integrity and RNA-binding capabilities of the NTD. By employing complementary techniques such as crystallography, solution NMR, SAXS, and other biophysical methods, we found that both aspects depend on a core network of residues conserved among Betacoronaviruses. The interactions within this expanded network significantly influence selective binding to target RNAs. We propose that this core network is essential for the protein’s stability and intrinsic flexibility, which are crucial in target RNA recognition.
_________________
O 08
Cutting-edge tools for structural biology: bringing AlphaFold and Foldseek to the people
Presenter: Christoph Elfmann (christoph.elfmann@uni-goettingen.de)
Authors:
Christoph Elfmann; Prof. Dr. Jörg Stülke
Göttingen University
Recently, AI-driven protein structure prediction using AlphaFold (1) has revolutionized biology. While tools like AlphaFold DB and AlphaFold Server have facilitated its use, the evaluation of prediction quality still presents challenges to users, particularly those that commonly work in the wet lab. We have developed PAE Viewer (https://pae-viewer.uni-goettingen.de) (2), a web tool for the interactive visualization of AlphaFold predictions and corresponding PAE (Predicted Aligned Error), describing the confidence in the relative positions of folds within the structure. Additionally, PAE Viewer supports the display of crosslinks, enabling the integration of experimental data. Structural homologies of proteins may indicate similar biological functions, despite a low sequence similarity. We integrated Foldseek (3) into SubtiWiki (https://subtiwiki.uni-goettingen.de) (4), a popular database for the model bacterium Bacillus subtilis, with the aim to make the search for structural homologs as simple as BLAST searches. We hope that these tools will enable a wide group of scientists to delve into the analysis of protein structures and help to bring these cutting-edge algorithms into the spotlight of microbiological research.
(1) Abramson, J. et al. (2024) Nature 630:493-500
(2) Elfmann, C. et al. (2023) Nucleic Acids Res. 51:W404-W410
(3) van Kempen, M. et al. (2024) Nat Biotechnol. 42:243-246
(4) Elfmann, C. et al. (2025) Nucleic Acids Res. 53: D864-D870
_________________
O 09
Design and development of synthetic G-CSFR modulators
Presenter: Dr. Mohammad ElGamacy (mohammad.elgamacy@med.uni-tuebingen.de)
Authors:
Dr. Mohammad ElGamacy¹; Dr. Birte Hernandez; Prof. Julia Skokowa
¹ University Hospital Tuebingen
Recent research has demonstrated synthetic cytokine receptor ligands can modulate diverse signaling outcomes. This signaling diversity can greatly depart from the canonical signaling footprint of natural cytokines, opening new therapeutic possibilities beyond what recombinant cytokines could achieve. Our aim was thus to design a diverse set of ligands targeting the granulocyte-colony stimulating factor (G-CSFR), a critical regulator of immune and inflammatory responses. G-CSFR signaling is essential for sustaining granulocyte production, but also regulates several hematopoietic and inflammatory processes, where conversely, its dysregulation is a key driver of many neoplastic and metastatic transformations.
Here we report the design of ligands that can engage the receptors in different modes, with the aim of tapping into different parts of the modulation spectrum; resulting in full agonists, biased agonists, as well as antagonists. Although our designs’ structures and sequences were profoundly different from the native ligand, they were highly potent in controlling G-CSFR signaling, as evaluated in a variety of in vitro and in vivo models. These novel modulators were also hyper-thermostable, proteolytically resistant, and their experimental structures agreed with their respective design models with atomic accuracy. Building on these results, we are currently proceeding with the further preclinical development of these proteins.
_________________
O 10
Coupling of ribosome biogenesis and translation initiation in human mitochondria
Presenter: Anna Franziska Finke (franziska.finke@med.uni-goettingen.de)
Authors:
Anna Franziska Finke¹; Dr. Marleen Heinrichs¹; Dr. Shintaro Aibara²; Prof. Dr. Ricarda Richter-Dennerlein¹; Prof. Dr. Hauke Hillen¹
¹ Universitätsmedizin Göttingen; ² Max-Planck-Institute for Multidisciplinary Sciences
Mitoribosomes synthesize essential oxidative phosphorylation subunits, requiring dedicated chaperones, RNA methylating enzymes, and GTPases for assembly. Defects in this process cause severe mitochondriopathies, yet the molecular mechanisms remain poorly understood. Here, we use single-particle cryo-EM to characterize late-stage assembly intermediates of the human small mitoribosomal subunit (mtSSU).
In particular, we have generated a human cell line lacking a key mtSSU biogenesis factor reventing premature subunit association. Transient expression of an affinity-tagged variant of the factor allowed us to isolate native mtSSU assembly intermediates directly from human cells. Single-particle cryo-EM analysis of these intermediates enabled us to resolve three distinct mtSSU assembly states and their associated factors, elucidating their role in 12S rRNA maturation. Strikingly, we additionally observed four distinct mtSSU states resembling primed initiation complexes that contained an incompletely matured rRNA helix. This suggests that ribosome biogenesis and translation initiation are not mutually exclusive processive but can occur simultaneously.
The combination of genetic perturbation, endogenous purification and single-particle cryo-EM offers a powerful approach for studying complex molecular machineries with potential applications in both academia and industrial research.
_________________
O 11
Functional analysis of synthetic cytokine receptors and possible heterodimerisation for optimised intracellular signalling
Presenter: Karlo Franke (karlo.franke@hhu.de)
Authors:
Karlo Franke
Institute for Biochemistry and Molecular Biology 2, Heinrich-Heine-University Düsseldorf
Rising numbers of obesity and diabetes cases are bringing new treatment approaches to the fore. Cytokines such as IL-6 and CNTF are known to have positive effects on weight reduction, but are not usable in practice due to their strong side effects like metainflammation. Chimeric cytokines ‘Cytokimeras’ are combinations of different cytokines that enable the dimerisation of non-natural receptor combinations. Furthermore they do not have pro-inflammatory effects as they activate fewer cells due to their specific receptor requirements. For the design of cytokimeras AI tools like AlphaFold 2 were used to predict structure and binding. In our work, we aim to create a chimera that binds erythropoietin receptor (EPOR) and leukaemia inhibiting factor receptor (LIFR). The strong difference in their extracellular matrix domains and steric confirmation makes it difficult to create a ligand that leads to heterodimerisation.
To determine whether receptors generally can form heterodimers, we utilized our established synthetic cytokine receptor platform that allows us to exclude steric effects by stimulation with synthetic ligands. Using this system, we were able to demonstrate heterodimerisation and intracellular signalling for different receptor constitutions like EPOR and LIFR. We further improved the signalling of EPOR heterodimers with modified intracellular domains. This approach offers a novel framework for optimising cytokimera design with therapeutic potential.
_________________
O 12
Protein Design For and With Synthetic Cells
Presenter: Béla Frohn (bfrohn@biochem.mpg.de)
Authors:
Béla Frohn¹; Andrey Sysoev¹; Dr. Kareem Al Nahas¹; Prof. Dr. Shunshi Kohyama²; Prof. Dr. Petra Schwille¹
¹ Max Planck Institute of Biochemistry; ² University of Tokyo
Bottom-Up Synthetic Biology creates cell-like systems from a minimal set of functional modules, such as purified proteins, membranes and DNA. This facilitates the study of biological systems under extremely well-defined conditions, where every parameter is known and can be controlled. Here we show that these “synthetic cells” provide an ideal screening platform to test designed proteins for complex biological functions, i.e., functions that only arise from interaction with the environment. We present an experimental pipeline to combine protein production and screening in a single step, utilizing cell-free expression inside synthetic cells. This circumvents the need for protein purification and enables high-throughput characterization. As examples, we design and screen proteins capable of intracellular pattern formation, as well as proteins capable of large-scale membrane deformation. Together, protein design and synthetic cell science will allow the construction of entirely new, cell-like biological systems for medical applications and to understand fundamental principles of life.
_________________
O 13
Expanding the available structure and sequence space of PET degrading enzymes
Presenter: Andreas Gagsteiger; Onur Turak (andreas.gagsteiger@uni-bayreuth.de)
Authors:
Andreas Gagsteiger; Onur Turak; Prof. Dr. Birte Höcker
Universität Bayreuth
The enzymatic degradation of polyethylene terephthalate (PET) offers a sustainable solution for PET recycling. Over the past two decades, more than hundred PETases have been characterized, primarily exhibiting similar sequences and structures. Albeit recent protein engineering successes, the potential for more efficient degradation is still present and the available sequence space is far from being exhausted. To better differentiate between highly and poorly active PET‑hydrolases we established a tunable degradation platform which incorporates realistic substrates and is adaptable to different polyesters. It significantly simplifies comparison and characterization of novel enzymes and designed variants. Benefiting on a parallelized purification approach, many candidates can be analysed in parallel. Using our platform we discovered new halophilic PETases from the marine Halopseudomonas lineage. A high resolution crystal structure of one candidate reveals a unique active site composition and a lack of the canonical π-stacking clamp found in PETases so far. These differences result in further variations of loop structures surrounding the active site. We characterized these HaloPETases under different reaction conditions including varying salt concentrations, pHs and temperatures by our PET-coated microtiterplate assay. Based on our findings we propose to extend the conventional PETase classification system by the newly identified type III PETases.
_________________
O 14
Graph-Theoretical Analysis of Functional Changes in an Open and Closed Conformation of Complex I
Presenter: Dr. Florian Gisdon (florian.gisdon@bioinformatik.uni-frankfurt.de)
Authors:
Dr. Florian Gisdon; Prof. Christoph Welsch; Prof. Ina Koch
Goethe University Frankfurt
Protein complexes are functional modules in the hierarchy of the cellular organisation and can be partitioned into smaller substructures with specific biological functions. Structural variations or conformational changes might enable functionality by rearranging the functional substructures.
We aim to provide a computational approach to predict and analyse functional biological modules in protein complexes. The analysis of different variants of protein complexes allows us to relate structural variations with changes in the functional substructures. Our approach might be important for classifying different protein variants in the context of pathologies or for functional protein design.
We modelled an open and closed conformation of respiratory complex I as undirected, labelled graphs. The conformational change from an open to a closed state is associated with the activation of complex I. We partitioned the graphs using the Leiden clustering algorithm to compute structural modules and analysed vertex centralities. We observed a correlation of the computed structural modules with functional substructures and a change in the structural modules related to mechanistic features. Our approach is implemented in the Protein Topology Graph Library. We aim to provide a suitable method to automatically analyse the highly complex protein-structure data and the increasing number of large protein complexes.
_________________
O 15
Severe discrepancies between the newly determined crystal structure of the RNA aptamer B1m15 in complex with the bacterial repressor DasR and the AlphaFold3-predicted structure.
Presenter: Jan Hartmann (jan.hartmann@fau.de)
Authors:
Jan Hartmann¹; Marharyta Makarova¹; Dr Michael-Paul Vockenhuber PhD²; Sigrid Weiler¹; Claudia Egerer-Sieber¹; Prof. Dr. Beatrix Suess²; Prof. Dr. Yves A. Muller¹
¹ FAU Erlangen-Nuremberg; ² Technical University Darmstadt
Non-coding RNAs are important regulators of gene expression in all kingdoms of life. Consequently, RNA molecules have become prominent in synthetic biology, and small regulatory RNAs, riboswitches and ribozymes are being applied as regulatory devices in the design of synthetic genetic circuits and networks. RNA molecules that bind protein targets with extraordinarily affinity and specificity can be identified de novo by in vitro selection (SELEX, Systematic Evolution of Ligands by Exponential enrichment). These so-called aptamers can adopt defined three-dimensional structures and form well-defined interaction surfaces similar to those found in antibodies. We have recently determined the crystal structure of the SELEX-derived aptamer B1m15 in complex with the bacterial repressor DasR at 2.0 Å resolution. In the complex, two aptamer molecules bind to the dimeric DasR protein with a 2:2 stoichiometry. Each B1m15 molecule individually sequesters one DNA-binding head of DasR. Since the structure is not yet published, the structure has not been seen by AlphaFold3 during training of the AI engine. Hence, a detailed comparison between the experimental structure and an AlphaFold3 structure prediction might yield valuable insights into current limitations of AI-generated structure predictions of protein-nucleic acid complexes. Here we show that when running AlphaFold3 with a suggested 2:2 stoichiometry, the predicted complex bears little resemblance with the experimental structure.
_________________
O 16
Step by step - Engineer Trp Repressor to Walk in a Tandem Gait
Presenter: Olive Laprévote (olivier.laprevote@uni-bayreuth.de)
Authors:
Olive Laprévote¹; Prof. Paul Curmi²; Prof. Heiner Linke³; Prof. Birte Höcker¹
¹ Universität Bayreuth; ² University of New South Wales (UNSW) Sydney; ³ Lund University
Inspired by kinesin, myosin and dynein, we're trying to make protein molecular walkers. We chose DNA as the track, TrpR as the foot, and glycine-serine repeats as the legs. Here we present how motors based on these components could walk in a clocked or autonomous fashion, what new properties are needed to be factored in to achieve walking, and how far we are into engineering these.
_________________
O 17
A novel rotamer library for nucleic acids enables the design of protein-nucleic acid complexes with the protein design software MUMBO
Presenter: Marharyta Makarova (marharyta.makarova@fau.de)
Authors:
Marharyta Makarova¹; Dr. Martin T. Stiebritz¹; Prof. Dr. Beatrix Suess²; Prof. Dr. Yves A. Muller¹
¹ FAU Erlangen-Nuremberg; ² Technical University Darmstadt
Current AI-tools for designing macromolecules have been struggling with DNA and RNA structure prediction and design, as the amount of respective experimental data available for training is significantly lower than that of proteins. Therefore, developing alternative approaches remains of interest. MUMBO is a program that allows designing protein-protein interactions and protein-ligand binding pockets by means of side-chain packing algorithms, where alternative amino acids and their rotamers are generated from libraries on a fixed backbone and the most favourable ones are selected based on the lowest overall energy. In order to extend MUMBO capabilities to designing nucleic acids, a nucleotide rotamer library is needed. Based on statistical analysis of pseudorotational angles and dihedral angles of more than 175,000 nucleotides from experimental structures deposited with the PDB database, a discrete pseudorotational angle-dependent nucleotide-specific rotamer library was derived. We validated the library by rebuilding more than 20,000 nucleotides in a custom dataset and showed that it allows modelling nucleotides with accuracies even slightly better than for amino acids. The implementation of the novel nucleotide rotamer library in MUMBO enables modelling and designing DNA and RNA sequences on a fixed backbone together with protein-nucleic acid interaction interfaces.
_________________
O 18
Computational analysis of translation elongation factors
Presenter: Dr. Marina Parr (mar.ark.parr@gmail.com)
Authors:
Dr. Marina Parr¹; Alina Sieber²; PD Dr. Jürgen Lassak²; Prof. Dr. Dmitrij Frishman¹
¹ Technische Universität München (TUM); ² Ludwig-Maximilians-Universität München
The efficient translation of proteins is essential for cellular function, yet ribosomal pausing at stalling motifs within mRNA sequences can disrupt this process. In bacteria, Elongation Factor P (EF-P) alleviates such pauses by promoting peptide bond formation. EF-P undergoes species-specific post-translational modifications, such as lysylation or rhamnosylation, at the tip residue of its β3Ωβ4 loop. This study investigates the co-evolution of EF-P sequences and their corresponding modification systems, revealing a conserved sequence pattern surrounding the modified residues in the β3Ωβ4 loop.
We also characterize EfpL, a poorly understood EF-P-like protein, which features an extended β3Ωβ4 loop containing a conserved arginine at the tip and a proline three residues upstream. Ribosome profiling in E. coli demonstrates that EfpL alleviates ribosomal pausing at distinct stalling motifs compared to EF-P. Identified as an assistant translation elongation factor, EfpL may represent a novel regulatory mechanism in translation dynamics, attributed to the presence of a conserved acylation site.
Together, these findings advance our understanding of how EF-P and EfpL mitigate ribosomal stalling, highlighting their roles in maintaining efficient protein synthesis.
_________________
O 19
Visual dysfunction caused by mutations in the cGMP-signaling protein complex
Presenter: Lars-oliver Peters PhD (lars-oliver.peters@uni-oldenburg.de)
Authors:
Lars-oliver Peters PhD
Carl von Ossietzky Universität Oldenburg
Guanylate cyclase-activating protein 1 (GCAP1) plays a crucial role in regulating retinal photoreceptor function by modulating guanylate cyclase (GC) activity in response to intracellular calcium (Ca²⁺) levels. At high Ca²⁺ concentrations, GCAP1 inhibits GC, while at low Ca²⁺ concentrations, it activates GC to restore cyclic GMP (cGMP) levels, ensuring proper phototransduction recovery. Mutations in GUCA1A, the gene encoding GCAP1, and GUCY2D encoding human GC-E disrupt this regulation and cGMP synthesis. They are implicated in inherited retinal diseases such as retinitis pigmentosa (RP) and cone-rod dystrophy (CRD), leading to progressive vision impairment. We investigated the biochemical and functional impact of a newly identified GCAP1 R93C variant, which was recently associated with RP. The R93C mutation is located in the EF3-hand motif of GCAP1, a critical region for calcium binding and subsequent regulation of retinal GC-E activity. Typical features of mutations in GCAP1 and GC-E are changes in calcium sensitivity by shifting the IC50 of GC inhibition to higher calcium concentrations. These shifts result in reduced calcium sensitivity and constitutive activation of GC, impairing phototransduction and contributing to retinal degeneration. We hypothesize a similar dysregulation of R93C reducing the ability of GCAP1 to effectively control cGMP synthesis and maintain cGMP-calcium homeostasis. We discuss potential structural alterations in GCAP1 arising from the mutation.
_________________
O 20
Enzymes for Polyurethane Degradation: Computational and Experimental Strategies for Design and Discovery
Presenter: Agata Raczyńska (agata.raczynska@polsl.pl)
Authors:
Agata Raczyńska¹; Dr. Oksana Kovalenko¹; Katarzyna Szleper¹; Dr. Tadeusz Bieg¹; Dr. Jeremy Esque²; Dr. Rajendra Sharma; Dr. Katarzyna Papaj¹; Marzena Szawara¹; Kinga Plasa¹; Dr. Anna Kasprzycka¹; Dr. Isabelle André²; Prof. Artur Góra³
¹ Silesian University of Technology, Gliwice, Poland; ² Toulouse Biotechnology Institute, TBI, Université de Toulouse, CNRS, INRAE, INSA, Toulouse, France; ³ Tunneling Group, Biotechnology Centre, Silesian University of Technology, Gliwice, Poland
Polyurethanes (PURs) are synthetic polymers resistant to natural degradation, contributing to environmental pollution. This study addresses key challenges in enzymatic PUR degradation through three objectives: defining PUR substrate diversity, investigating molecular determinants of enzymatic activity, and discovering novel PUR-degrading enzymes.
To explore PUR diversity, we developed PUR-GEN, a web server that automates the generation of PUR fragment libraries, enriched with conformers and physicochemical properties. Molecular docking of 414 generated oligomers demonstrated PUR-GEN’s utility in streamlining enzyme selection for biodegradation.
Next, we analysed Thermobifida fusca cutinase (TfCut2) as a model enzyme for PUR degradation. Computational studies elucidated key substrate interactions and guided rational design of TfCut2 mutants with enhanced binding. These predictions were validated experimentally, linking in silico findings with enzyme performance.
Finally, metagenomic sequencing of the Marmara Sea microbiome led to the identification of novel candidate enzymes for PUR degradation. Bioinformatics screening and experimental validation revealed promising biocatalysts, highlighting untapped ecological potential in tackling plastic pollution.
This study integrates computational tools, enzyme engineering, and bioprospecting to advance the discovery and optimisation of PUR-degrading enzymes.
_________________
O 21
Quantitative analysis of Ezrin mutants defective in f-Actin Binding observed with in vitro minimal Actin cortices
Presenter: Johanna Rathke (Jrathke@posteo.de)
Authors:
Johanna Rathke; Elena Ackermann; Prof. Dr. Claudia Steinem
Georg August University Göttingen, Germany
Actin as a polymeric component of the cytoskeleton is crucially involved in many physiological processes including cell motility, mitosis and remodelling morphology. In the periphery of the cell, attached to the membrane, actin occurs as a highly structured dynamic network, the actin cortex. To ensure its dynamic, various actin-binding proteins are involved in the formation of the cortex, including membrane-actin-linker, enabling the attachment to the plasma membrane. Ezrin plays a crucial role ensuring the cortex dynamic by facilitating the direct binding to the membrane via PtdIns[4,5]P₂. In this work, we focus on deciphering the direct f-actin-ezrin binding sites, since the exact binding mechanism is still elusive. To comprehend these interaction the formation of minimal actin cortices on solid-supported lipid bilayers is used as a bottom-up approach to represent the plasma membrane in a controllable environment, reduced in complexity. Point mutants of ezrin‘s predicted actin binding site and non-muscle actin are used to process the cortex formation that is observed by confocal laser scanning microscopy. To quantify the effect of the substituted amino acids for the f-actin-ezrin attachment a virtual reconstruction of the individual actin filaments will be applied.
_________________
O 22
Functional and structural characterization of UbiB
Presenter: Johannes Regner (johannes.regner@bct.uni-halle.de)
Authors:
Johannes Regner; Julian Ühlein; Dr. Lars Franke; Prof. Dr. Frank Bordusa; Prof. Dr. Hauke Lilie; Dr. Constanze Breithaupt; Dr. Christoph Parthier; Prof. Dr. Milton T. Stubbs
Martin-Luther-Universität Halle-Wittenberg
The final six steps of the ubiquinone biosynthesis in E. coli are catalyzed by a soluble metabolon, involving at least seven proteins [1]. As knockout mutants of ubiB accumulate octaprenylphenol (OPP) [2], UbiB may be involved in extracting the initial substrate OPP from the membrane or in the formation of the metabolon, although this has not yet been proven [3]. We cloned a truncated, soluble construct of EcUbiB and performed ATPase activity assays of the purified enzyme in the absence and presence of substrate analogues that mimic OPP.
[1] M. Chehade et al. A Soluble Metabolon Synthesizes the Isoprenoid Lipid Ubiquinone Cell Chem Biol 2019, 26 (4), 482-492
[2] W. Poon et al. Identification of Escherichia coli ubiB, a Gene Required for the First Monooxygenase Step in Ubiquinone Biosynthesis J Bacteriol. 2000, 182(18), 5139-5146
[3] A. Reidenbach et al. Conserved Lipid and Small-Molecule Modulation of COQ8 Reveals Regulation of the Ancient Kinase-like UbiB Family Cell Chem Biol 2018, 25(2), 154-165
_________________
O 23
Exploring the gas channel in [FeFe] hydrogenases
Presenter: Prof. Dr. Ingrid Span (ingrid.span@fau.de)
Authors:
Prof. Dr. Ingrid Span
Friedrich-Alexander-Universität (FAU) Erlangen-Nürnberg
Hydrogen gas is an environmentally sound energy carrier with high energy density. Hydrogen is not a natural resource and current methods to produce hydrogen rely mostly on fossil fuels. A sustainable method for hydrogen production would be a major advance towards a carbon-neutral society. Hydrogenases are highly efficient enzymes that catalyze the reversible reaction of protons and electrons to molecular hydrogen. Among the different classes of enzymes, the [FeFe] hydrogenases are highly active and biased towards the production of molecular hydrogen. The incredible efficiency of hydrogen-producing enzymes derives from the fine-tuned active site clusters that enable fast catalysis and the protein environment that enables efficient transport of substrates and products between the active site and protein surface. We have used high pressure freezing techniques to map the gas channel between active site and protein surface by derivatizing hydrogenase crystals with krypton gas. The visualization of the gas channel provides important insights into how the produced hydrogen gas is transported through the protein environment and opens exciting possibilities for modulating its properties by protein engineering.
_________________
O 24
Analysis of Interactions between the γ-Tubulin Ring Complex with the Centrosomal Proteins CDK5RAP2 and CP148 in Dictyostelium discoideum
Presenter: Pascal Vielberth (pascal.vielberth@student.uni-luebeck.de)
Authors:
Pascal Vielberth
University of Lübeck
The model organism Dictyostelium discoideum (D. discoideum) has an acentriolar centrosome that is commonly subdivided into the corona, the outer and inner core layers. The corona proteins CDK5RAP2 and CP148, two γ-tubulin complex (γ-TuC) scaffolding proteins, are of vital importance for the correct organisation of the centrosomal corona. CDK5RAP2, is known to interact with Cep192 and CP248. γ-TuCs consist of γ-tubulin, Spc97 and Spc98 in D. discoideum. To further analyse interacting proteins of CDK5RAP2, CP148 and γ-tubulin, yeast two-hybrid screens as well as BioID proximity labelling experiments were performed. BioID experiments revealed the proximity of CDK5RAP2 to CP148, Cep192, Spc97 and probably γ-tubulin but not of Spc98, indicating that Spc98 is located further away in the γ-TuC. CP148 is also in proximity of Cep192 and Spc97. Furthermore, Spc97 and Spc98 are shown to be within the γ-tubulin-BioID2 labelling radius. Thus, γ-tubulin is probably located between Spc97 and Spc98. This further indicates that γ-TuCs are anchored to CDK5RAP2 and possibly also to CP148.
The observed proximities from BioID experiments were compared to known interactions for orthologous proteins in Schizosaccharomyces pombe (S. pombe). Although mere proximity of proteins does not demonstrate their interaction, most reported interactions between analysed proteins are corroborated by proximity labelling BioID experiments performed.
_________________
O 25
Non-canonical G protein signaling in magnetoreception of European robin
Presenter: Srdan Vujinovic (srdjan_vujinovic@hotmail.com)
Authors:
Srdan Vujinovic¹; Ümmügülsüm Güzelsoy; Dr. Alexander Scholten; Dr. Rabea Bartölke; Prof. Dr. Karl-Wilhelm Koch
¹ C.v.O. University of Oldenburg
Heterotrimeric cone-specific G-proteins (Gt) are molecular switches, essential for intracellular signaling and are proposed to mediate magnetoreception in European robins via interaction with cryptochrome 4a (ErCry4a), a putative magnetoreceptor in the retina. While the α- and γ-subunits of Gt are suggested to interact with ErCry4a, the downstream signaling pathway leading to an electrophysiological response remains unknown. Effective magnetoreception likely depends on a fixed orientation of ErCry4a, which may be facilitated by the lipid modifications of Gt subunits anchoring them to membranes.
We studied the interaction of Gt subunits with ErCry4a and immobilized lipid bilayers using surface plasmon resonance (SPR). As such, the α-subunit was expressed and purified in E. coli, while the βγ-complex was produced in Sf9 cells via the baculovirus expression system. Prior studies demonstrated high-affinity binding between Gtα and ErCry4a without lipids. Here, we found that the myristoylated α-subunit exhibited greater affinity for immobilized liposomes on the SPR chip compared to the non-lipidated form. Additionally, the presence of Gt subunits altered the mode of ErCry4a interaction with lipid bilayers. These findings suggest that lipid-modified Gtα enhances membrane association and may stabilize ErCry4a orientation, aiding magnetic field sensing. Future work will explore the role of Gtβγ in forming functional complexes with ErCry4a to better understand avian magnetoreception.
_________________
O 26
Elucidating the structural basis for phenotypical variations caused by naturally occurring YUCCA8 proteoforms in Arabidopsis thaliana
Presenter: Nex Maris Winkler (nex-maris.winkler@biochemtech.uni-halle.de)
Authors:
Nex Maris Winkler¹; Dr. Constanze Breithaupt¹; Dr. Ricardo Fabiano Giehl²; Duc Anh Nguyen¹; Yukti Yukti²; Prof. Dr. Nicolaus von Wirén²; Prof. Dr. Milton T. Stubbs¹
¹ Martin Luther University Halle-Wittenberg; ² Leibniz Institute of Plant Genetics and Crop Plant Research (IPK)
The YUCCA (YUC) family of flavin-dependent monooxygenases (FMO) is involved in the biosynthesis of the phytohormone Indole-3-acetic acid in plants. Jia et al. identified two single nucleotide polymorphisms in yuc8 that show a stark difference in root development under low nitrogen conditions [1]. The corresponding proteoforms differ in a single amino acid not known to be involved in the catalytic activity. YUC8 is membrane associated FMO [1], and based on the properties of mammalian FMOs [2], the membrane association of YUC8 is hypothesized to be mediated through a hydrophobic alpha helix. Based on a multiple sequence alignment with cytosolic members of the YUC family, we mutated two residues in this helix. The double mutant could be produced recombinantly in E. coli, opening up the possibility for enzymatic characterization and structure determination to elucidate the molecular basis for the differences in root foraging. Additionally, the proteoforms will be further studied in planta.
[1] Jia, Z. Et al., Nat. Commun., 2021, 12, 5437. https://doi.org/10.1038/s41467-021-25250-x
[2] Bailleul, G. Et al., J. Biol. Chem., 2021, 296, 100221. https://doi.org/10.1074/jbc.RA120.016297
_________________
P 01
Decoding homeostatic pathways: from data Streams to molecular mechanisms
Presenter: Dr. Ramachandra M Bhaskara (bhaskara@med.uni-frankfurt.de)
Authors:
Dr. Ramachandra M Bhaskara
Goethe - Universität Frankfurt
Autophagy, protein ubiquitination, and sumoylation form the three central pillars of cellular homeostasis. In light of recent advances in omics and HTP experiments, data-driven research using inductive reasoning provides a key advantage, leveraging the immense volume of biological data. By combining modeling and simulation workflows with data science approaches, we integrate disparate biological datasets to gain molecular insights into these pathways. (1) We showcase a metric learning approach for the multiscale classification of the human E3 ligome. (2) From protein content profiling of autophagosomes, we identify potential selective autophagy receptors (SARs), expanding the landscape of known SARs. (3) Our molecular modeling and simulations show how PTMs and IDRs modulate the kinetics of RHD-mediated spontaneous budding to amplify ER-phagy. By modeling membrane proteins as anisotropic inclusions with distance-dependent non-zero spontaneous curvature, we capture their essential intrinsic curvature properties, laying the groundwork for mesoscale modeling of the autophagic process.
_________________
P 02
gapTrick – Structural characterisation of protein-protein interactions using AlphaFold with multimeric templates
Presenter: Dr. Grzegorz Chojnowski PhD (gchojnowski@embl-hamburg.de)
Authors:
Dr. Grzegorz Chojnowski PhD
EMBL Hamburg
The structural characterisation of protein-protein interactions is a key step in understanding the functions of living cells. Models of protein complexes provide important insights into interaction mechanisms, but their interpretation can be difficult if they lack fine structural detail, which is often the case in experimental and predicted models.
I will show that AlphaFold3 [1] often fails to predict protein complexes that are either weak, or dependent on the presence of a cofactor that is not included in a prediction. To address this problem, I developed gapTrick [2], an AlphaFold2-based approach that uses multimeric templates to improve prediction reliability. The tool uses AlphaFold2 [3] neural network models trained on single-chain proteins, which helps to avoid training set bias and allows for an extensive validation using a large benchmark set of protein complexes.
I will show that gapTrick improves the prediction of weak and incomplete complexes based on templates of low accuracy. I will present examples of gapTrick applications to the interpretation of difficult cryo-EM maps. I will also show that gapTrick can identify crucial residue-residue interactions in cryo-EM models, which would otherwise require laborious techniques such as deep mutational scanning.
The gapTrick code and Colab notebook are available at https://github.com/gchojnowski/gapTrick
[1] Abramson, J., et al. Nature 2024:1--3
[2] Chojnowski, G. biorxiv 2025
[3] Jumper, J., et al. Nature 2021;583--58
_________________
P 03
Mapping of allosteric protein landscapes using AI approaches
Presenter: Dr. Sandrine Coquille (sandrine.coquille@ibs.fr)
Authors:
Dr. Sandrine Coquille; Dr. Dominique Madern
CNRS - IBS
The lactate/malate dehydrogenase (LDH/MalDH) superfamily is a particularly interesting model for studying the emergence and evolution of allostery. Indeed, it offers a large diversity of homologs presenting a vast variety of pre-allosteric and allosteric behaviors. Using evolution-guided point mutations, X-ray crystallography and molecular dynamics simulations, we got insights on how allosteric contact networks were established during evolution and on the mechanisms linking non-allosteric to allosteric enzymes at the molecular level.
AI-based approaches may provide further perspectives to our project, notably in the identification of allosteric binding sites and hidden allosteric pockets available in meta-stable intermediate states of these enzymes. Small and hidden binding pockets are usually difficult to detect using traditional methods such as X-ray crystallography and NMR spectroscopy. It will be then interesting to investigate how the protein dynamics is modulated by the binding of allosteric effectors with potential therapeutic interests.
_________________
P 04
Assessing AI Driven Protein Structure Prediction Methods with Heparanase 2 Splicing Forms
Presenter: Jacqueline Krohn (krohn.jacqueline@mh-hannover.de)
Authors:
Jacqueline Krohn; Prof. Dr. Dietmar J. Manstein
Hanover Medical School
Heparanase 2 (Hpa2) is an enzymatically inactive isoform of Heparanase 1 (Hpa1), an enzyme critical for degrading heparan sulfate proteoglycans (HSPGs) and a prominent target in cancer therapy. Hpa2 exists in three alternative splicing forms – Hpa2a, Hpa2b, and Hpa2c – with Hpa2c being the full-length protein containing an untruncated TIM-barrel domain. Hpa2c shares 41% sequence identity and 56% similarity with Hpa1, providing a basis for constructing a reliable reference homology model (rHM) using Prime 7.6. Predicted models for Hpa2c, utilizing tools such as AlphaFold 3 (AF3) and Chai-1, exhibited substantial consistency with the rHM, yet exhibited variability in their flexible regions, particularly in the top region of the TIM-barrel (residues 130–200). Hpa2b introduced additional challenges due to its more extensive sequence compared to Hpa2a, necessitating the development of a novel template. Prediction reliability, as indicated by pTM scores, decreased further when predictions were restricted to the TIM-barrel sequence alone. Each AI-driven method demonstrated unique strengths: Chai-1 predictions without multiple sequence alignments (MSA) produced compact models, while MSA-based Chai-1 predictions achieved the highest pTM scores. AF3 models showed the closest resemblance to the rHM. In conclusion, the Chai-1 models generated using MSA appear to be the most realistic.
_________________
P 05
Beating viruses at their own game: exploiting structural homology and protein structure predictions to identify new viral host-virus protein-protein interactions.
Presenter: Pasquale Lamagna (pasquale.lamagna@leibniz-liv.de)
Authors:
Pasquale Lamagna¹; Sanjana Nair²; Mathias Pfeifer²; Emilia Wassiljew²; Marcus Altfeld³; Maya Topf²
¹ LIV/CSSB; ² Centre for Structural Systems Biology (CSSB); ³ Department of Integrative Virology, Leibniz-Institut für Virologie (LIV)
Viruses are infamously efficient at employing homology as a mechanism of immune evasion by imitating host proteins or molecular patterns to escape immune recognition and response. Recent advancements in bioinformatics have made it possible to identify evolutionary relationships between distant proteins, including those found in viruses and their hosts. These common evolutionary patterns often manifest themselves in similar structural folds, which might be
utilized to identify and model new virus-host interactions. Based on this concept, we have implemented a computational workflow to identify potential virus-host protein-protein interactions that may modulate the host immune response, across all viral taxonomies. Applied on the genome of Human Cytomegalovirus (HCMV) we conducted structural homology search using FoldSeek (1) to list viral proteins and their homologs in humans. Matched human proteins were
filtered for immune-related functions and their interacting partners were collated from literature. Finally, we used AlphaFold3 (2) to predict structures of putative complexes between the immune-mimicking viral proteins and their respective human counterparts. Complexes predicted at high quality are validated through in vitro functional studies. By characterizing the interaction of these proteins, we hope to shed light on new immune evasion mechanisms employed by HCMV
and other viruses.
References:
1. van Kempen et al., nature biotechnology, 2024
2. Abramson et al., nature 2024
_________________
P 06
Structural annotation of disease-relevant protein interaction interfaces involving disorder
Presenter: Dr. Katja Luck PhD (k.luck@imb-mainz.de)
Authors:
Dalmira Hubrich PhD; Jesus Alvarado Valverde; Chop Yan Lee PhD; Milena Djokic; Mareen Welzel; Dr. Katja Luck PhD¹
¹ Institute of Molecular Biology (IMB)
Protein-protein interactions (PPIs) are being mapped experimentally at increasing throughput. However, HTP PPI assays can only tell us which proteins interact with each other but not how. This lack in structural information massively hinders inference of physiological and pathological molecular mechanisms. The structural diversity by which proteins can interact with each other is immense and largely driven by disordered regions in proteins that mediate transient signaling and regulatory PPIs. Many identified disease-associated human variants fall in disordered protein regions and remain uncharacterized partially because lack of explicit sequence conservation hinders their effect predictions. Structures of PPI interfaces involving disordered regions would advance variant effect prediction, however, we find that computational tools such as AlphaFold struggle to accurately predict these modes of protein binding. Using our developed pipelines we generate high confident structural models of 3500 disorder-containing PPI interfaces and identify close to 2700 uncharacterized mutations in disordered regions predicted to mediate protein binding. We experimentally validate selected predicted PPI interfaces and confirm PPI perturbation for several tested variants. Our work delineates a highly promising integrative structural biology approach towards advancing functional annotations of disordered regions in proteins, improving variant effect predictions and deciphering disease mechanisms.
_________________
P 07
Integrating Artificial Intelligence Based and Classical Structural Biology Methods to Understand the Impact of the β-Actin G74S Mutation on Histidine Methylation
Presenter: Anja Marquardt (marquardt.anja@mh-hannover.de)
Authors:
Anja Marquardt; Prof. Dr. Dietmar J. Manstein
Hannover Medical School (MHH)
Single-point mutations in cytoplasmic actin isoforms are associated with diseases such as Baraitser-Winter Cerebrofrontofacial Syndrome (BWCFF). While these mutations disrupt actin’s biochemical functions, their molecular mechanisms remain unclear. Here, we investigate the b-actin G74S mutation, which causes severe BWCFF. This mutation occurs in actin’s sensor loop (a.a. 70-78), a region critical for nucleotide binding and protein interactions. Recombinant mutant actin exhibits reduced His73 methylation and alterations in thermal stability, nucleotide exchange, and polymerization. To understand these effects, we analyzed the methylation of the sensor loop peptide by SETD3. In silico mutagenesis predicts steric clashes with the mutant, and classical docking shows only the wildtype His73 positioned correctly in the methylation pocket, indicating significant structural rearrangements are required for SETD3 to accommodate the mutation. AI-based tools provide further insights: AlphaFold-3 predictions closely resemble the wildtype structure with minor local adjustments, while Chai-1 generates diverse models positioning His73 near the catalytic site, requiring structural adaptations to enable methylation. Both approaches reveal the peptide’s flexibility and the enzyme’s adaptability, consistent with reduced methylation efficiency. These findings demonstrate the utility of integrating AI-driven tools to elucidate structural and functional impacts of disease-linked mutations.
_________________
P 08
AlphaFold3-based Prediction and Design of GPCR-G protein Coupling
Presenter: Pasquale Miglionico (pasquale.miglionico@sns.it)
Authors:
Pasquale Miglionico; Marin Matic; Prof. Francesco Raimondi
Scuola Normale Superiore, Pisa
GPCRs are crucial cell signaling regulators that mediate extracellular stimuli via selective G-protein coupling. Limited experimental GPCR-G protein structures hinder our mechanistic understanding of this selectivity. To address this, we performed comprehensive structural modeling of human GPCR-G protein complexes using AlphaFold3, generating a complete structural atlas. The analysis of these predicted structures showed a significant correlation between AlphaFold quality metrics (e.g., pLDDT, interface pTM) and experimentally validated GPCR-G protein coupling specificity. Leveraging these metrics, alongside Rosetta-computed interface energy, we trained a TabPFN classifier. This classifier effectively discriminates coupled from uncoupled pairs with approximately 75% accuracy, demonstrating that AlphaFold can be used to predict the specificity of GPCR-G protein binding. Validated against known couplings from the IUPHAR database, our model successfully predicts novel couplings. This tool is particularly useful to better understand the signaling of under-characterized GPCRs like olfactory receptors, providing experimentally testable hypotheses on their biological role. Beyond prediction, we are applying this approach, together with a tree search algorithm based on ProteinMPNN, to the design of GPCRs that specifically bind a given G protein, showing the applicability of our method to the manipulation of GPCR signaling pathways.
_________________
P 10
Pathogenic potential of RalGAP variants explained by combined structural and computational analysis
Presenter: René Rasche (r.rasche@uni-muenster.de)
Authors:
René Rasche¹; Dr. Björn U. Klink²; Dr. Lisa H. Apken³; Esther Michalke³; Dr. Andrea Oeckinghaus⁴; Prof. Dr. Christos Gatsogiannis²; Prof. Dr. Daniel Kümmel Daniel¹
¹ Institute of Biochemistry, University of Münster ; ² Institute of Medical Biophysics and Center for Soft Nanosciences, University of Münster ; ³ Institute for Molecular Tumor Biology, University of Münster; ⁴ Institute for Molecular Tumor Biology, Unive
The small GTPases RalA and RalB control proliferation and regulate endocytosis and exocytosis. Imbalanced Ral-signaling promotes severe forms of cancers and is related to oncogenic Ras signaling. RalGAP complexes are potent tumor suppressors and crucial to limit Ral as well as Ras signaling. The human RalGAP complexes consist of one of two RalGAPα subunit isoforms and a common RalGAPβ subunit. RalGAP differs from homologous Asn-thumb GAP domains due to the necessity of heterodimerization for functionality. We combined cryo-EM and computational structural biology to provide a model of the large 580 Å moustache shaped RalGAP2 complex and its substrate Ral [1]. We identified and characterized novel interaction domains that mediate complex assembly and found that the unique architecture of RalGAP is required for its functionality in vivo. To assess a possible pathogenic function of reported RalGAP variants of unknown clinical significance (VUS) from cancer patients, we evaluated the AI-driven classification by AlphaMissense based on our experimental structure. Predictions very well identify mutants that impair secondary structure but are less accurate in detecting those that affect complex assembly and interaction interfaces. Thus, in context of protein complexes, structure-guided analysis of VUS provides a more comprehensive understanding of possible pathogenicity than automated characterization alone.
[1] Rasche, R.; Klink, B.U et al. (2024) bioRxiv 2024.11.25.625123
_________________
P 11
FRET-assisted structural modeling of dynamic protein ensembles
Presenter: Bianca Reschke (bianca.reschke@hhu.de)
Authors:
Bianca Reschke; Dr. Christian A. Hanke; Dr. Alexander Larbig; Prof. Dr. Claus A. M. Seidel
Heinrich-Heine-Universität Düsseldorf
Förster Resonance Energy Transfer (FRET) experiments provide information on the structure, dynamics and conformational transitions of biomolecules. FRET experiments combined with computational methods in integrative approaches allow the generation of dynamic ensembles and the resolution of static and dynamic heterogeneities with high accuracy and precision. The use of single-molecule FRET measurements with a diffusion-based confocal microscope and FRET observables together with molecular simulations and FRET-restrained structural modeling allows screening and scoring of an ensemble of conformations against experimental FRET data to identify conformers with the best agreement with experimental data. Integrative structural models obtained from various experimental data can be deposited in the archiving system PDB-Dev.
One system of interest is the carbohydrate-binding module 56 (CBM56), a C-terminal subunit of the Glucan endo-1,3-D-glucosidase from Bacillus circulans. As a target protein for the CASP 13 competition, its structure has not yet been solved experimentally by X-ray crystallography. The structure proposed by homology modeling consists of two domains composed mainly of beta-sheets, connected by a linker unit.
_________________
P 12
Integration of experiment and theory unveils structure, dynamics, and function of multi-protein complexes
Presenter: Prof. Dr. Till Rudack (till.rudack@ur.de)
Authors:
Torben Fürtges; Elias Wippermann; Prof. Dr. Till Rudack
University of Regensburg
The cryo-EM resolution revolution initiated the rise of structural investigations of multi-protein complexes. The recent AI-driven structure prediction breakthrough headed by AlphaFold dramatically facilitated the process to obtain structural models of such complexes. Exploiting these advances, we established the here presented integrative modeling strategies combining molecular dynamics (MD) simulations and AI driven structure prediction with data from structural biology (ranging from NMR to cryo-ET), biophysical, and biochemical experiments. With these strategies, we predict experimentally validated structural dynamic models of multi-protein complexes. We derived hypothesis about molecular mechanism based on protein interaction networks identified by our developed tool PyContact. Such insights drive targeted design of experiments e. g. by suggesting mutagenesis studies of the key functional regions of the identified networks. Applying our strategy, we discovered molecular mechanisms of protein degradation by the proteasome, RNA degradation by the exosome, and assembly of photosystem II.
Notably, in some cases of our collaborative studies the predictions matched the experimental structures perfectly while in other cases there were significant deviations, mostly due to prediction issues. This uncertainty highlights the need of reliability measures, further structure assessment tools, and validation strategies for predicted structural models.
_________________
P 13
Challenges for AI driven structure prediction: The peacock's feather keratin
Presenter: Peter Russ (peter.russ@bio.uni-stuttgart.de)
Authors:
Peter Russ¹; Helmut Kirchner; Herwig Peterlik; Ingrid Weiss
¹ University of Stuttgart (D)
The structure of F-keratin has puzzled scientists for nearly a century. Initial X-ray data obtained by Astbury and colleagues and later interpretations proposed by Fraser and Parry have defined our understanding of F-keratin’s architecture for decades. Despite recent advances in AI-driven models, a meaningful structure of F-keratin remains elusive. Through functional segmentation, we identified an "N-block" structure comprising four strands (AA 1–52) arranged in a twisted parallelepiped. Although promising due to its β-sheet core, this initial building block for the multimeric fiber alone did not satisfy all essential criteria from experimental data. Further analysis of the amino acid composition revealed a second block: the "C-block", a sandwich of four β-strands (AA 81–100). By fitting the tetrameric N- and C-blocks together, we developed a model that perfectly explains observed X-ray diffraction patterns. The remaining part, primarily small and flexible amino acids (GSA-string) forms an elastic cross-linking matrix with major implications for excellent mechanical features of F-keratin: flexibility & strength. This model resembles a nano-scale "vertebrate spine", with the N-block as vertebral bodies, the C-block as intervertebral discs, and the GSA-string as surrounding ligaments. During structure formation, the "spine" tilts similarly to scoliosis, but unwinds under pressure into a fiber-reinforced composite with an axial periodicity of 9.5 nm.
_________________
P 14
Model of the inactive dimeric conformation of TAp63α
Presenter: Büsra Yüksel (busr.yuksel@gmail.com)
Authors:
Büsra Yüksel¹; Dr. Sonja Welsch²; Janet Vonck²; Prof. Dr. Volker Dötsch³
¹ IMPRS, Max Planck Institute for Biophysics; Goethe University; ² Max Planck Institute of Cellular Biophysics; ³ Goethe University Frankfurt and Center for Biomolecular Magnetic Resonance (BMRZ)
TAp63α is an isoform of the transcription factor p63 that is found in oocytes when they are arrested in prophase of meiosis I. In these resting oocytes TAp63α is kept in an inactive and only dimeric conformation. Upon the detection of DNA damage, in particular DNA double-strand breaks, TAp63α gets phosphorylated by CHK2 in a loop N-terminal to the transactivation inhibitory domain (TID). This modification does not change the conformational state of TAp63α, but recruits another kinase, CK1. Phosphorylation by CK1 leads to the disruption of the inhibitory mechanism and, the formation of a transcriptionally active state and the induction of apoptosis. The inactive dimeric state of TAp63α is a kinetically trapped high-energy state, while the active tetrameric one is energetically more stable. We want to determine the structure of the closed dimeric state and had previously proposed that sequences in the N-terminus in combination with the C-terminal TID form a six-stranded β-sheet that blocks the tetramerization interface of the oligomerization domain, thus keeping TAp63α in a dimeric state. To achieve a high-resolution structure of dimeric TAp63α, we are using negative stain EM, Cryo-EM, biochemical methods as well as Alphafold3 prediction models to further characterize the TAp63α dimer for understanding activation mechanism.
_________________
P 15
Analytical high-throughput assays for generation of validated datasets to train ML models for cellulase optimization
Presenter: Dr. Simon Straß (simon.strass@nmi.de)
Authors:
Dr. Simon Straß¹; Sandra Maier¹; Philipp Schellenberger²; Lenz Lorenz²; Dr. Sven Benson²; Dr. Anne Zeck¹
¹ NMI Natural and Medical Sciences Institute at the University of Tübingen; ² Candidum GmbH
The efficient enzymatic hydrolysis of cellulose is essential for numerous biotechnological applications, including recycling and biomass conversion. However, predicting cellulase activity on different substrates remains challenging due to the interplay between enzyme structure, stability, and binding/catalytic function. Here, we present an experimental workflow for generating datasets that can serve as the foundation for ML-based activity predictions. While our dataset is still in development, preliminary data have been collected.
Our approach starts with a diverse library of cellulases which are tested in enzymatic assays to determine cellulose degradation, yielding kinetic parameters that serve as a functional benchmark. These cellulases are subjected to proteolytic digestion (e.g., Papain) to separate catalytic and carbohydrate-binding domains, as well as enzymatic deglycosylation. Intact-MS analysis before and after enzymatic digestion provides insights into molecular weight shifts and glycosylation status. SEC enables the characterization of oligomerization states and aggregation tendencies, while nanoDSF determines thermostability and pH optimum.
By integrating structural and functional datasets, we provide a robust framework that facilitates the correlation of biophysical properties with enzymatic performance. As we continue to expand our dataset, we aim to establish a thorough resource for the scientific community to develop ML models for cellulase activity prediction.
_________________
P 16
Modelling conformational transitions in macromolecular interactions
Presenter: Prof. Dr. Milton T. Stubbs (stubbs@biochemtech.uni-halle.de)
Authors:
Prof. Dr. Milton T. Stubbs
Martin- Luther- Universitaet Halle- Wittenberg
The intrinsically disordered protein (IDP) Gab1 (Grb2-associated binder 1) binds a multitude of important signaling proteins through its long disordered C-terminal tail [1], acting as an intracellular hub to orchestrate extensive signaling pathway cross-talk [2]. We recently determined the structure of a bidomain of the protein phosphatase SHP2 (PTPN11) in complex with the bis-phosphorylated Gab1 peptide pY627pY659-Gab1613-694 using microED [3]. The structure reveals novel insights into the activation mechanism of SHP2 by Gab1, and in combination with comprehensive dynamic and thermodynamic data delivers a solid foundation for further advancements in our understanding of the role of SHP2 in cell signalling and its potential as a target for drug development. In this presentation, we explore the performance of AlphaFold 3 to predict conformational changes driven by complex formation.
References
[1] Wöhrle FU, Daly RJ, Brummer T, Cell Commun Signal 2009, 7: 22.
[2] Lewitzky M, Simister PC, Feller SM, FEBS Lett 2012, 586: 2740-2750.
[3] Machner L, Shaikhqasem A, Gruber T, Hamdi F, Breithaupt C, Kniest J, Wiebe F, Lewitzky M, Parthier C, Kyrilis FL, Balbach J, Kastritis PL, Feller SM, Stubbs MT, BioRxiv, doi: https://doi.org/10.1101/ 2024.12.23.630100
_________________
P 17
Moonlighting signal peptides of protein isoforms discovered by deep learning
Presenter: Tomer Tsaban (tomer.tsaban@mail.huji.ac.il)
Authors:
Tomer Tsaban
The Hebrew University of Jerusalem
Recent developments in computational biology have enabled structural and functional protein studies at an unprecedented scale. However, even highly accurate structure prediction methods like AlphaFold have their limitations. Protein splice isoforms represent one such challenge. These unique and low-abundance variants greatly expand the functional landscape of protein-coding genes, and their structure prediction remains difficult due to inherent biases towards the structures of “canonical” proteins (e.g. in multiple sequence alignments and homology-driven modeling).
Here, we characterized the structure of an active, antagonistic splice isoform of SLAMF6 and uncovered a novel functional mechanism involving a domain-stabilizing signal peptide. We then developed a pipeline to identify additional isoforms where signal peptides compensate for missing elements, and explored computational approaches to evaluate the reliability of their AlphaFold models. From a broader perspective, our findings provide insights into how isoforms may fold, and suggest means of studying irregular proteins by addressing AlphaFold “blindspots”.
_________________
T 01
BiochemicalAlgorithms.jl -- Rapid application development in Julia
Presenter: Jennifer Leclaire MSc. (leclaire@uni-mainz.de)
Authors:
Jennifer Leclaire MSc.; Dr. Thomas Kemmer; Prof. Dr. Andreas Hildebrandt
JGU Mainz, Institute of Computer Science
Software packages in structure-based bioinformatics are often specialized for a single application or a specific target audience. BiochemicalAlgorithms.jl addresses this gap by providing an open-source platform with rich functionality for several tasks in molecular structure analysis. Redesigned from the popular Biochemical Algorithms Library (BALL), it aims to serve as a rapid application development (RAD) platform in Julia. Julia’s growing popularity in scientific machine learning stems from its powerful differentiation capabilities. In molecular mechanics, this enables innovative approaches like implementing force fields as neural networks or dynamically tuning parameters.
BiochemicalAlgorithms.jl provides flexible interfaces for the integration of these custom implementations and serves as a starting point for structural analysis by offering comprehensive functionalities:
- Data handling by reading common data formats such as PDB, PDBx/mmCIF, HyperChem HIN, SDF, and PubChem JSON
- Preprocessing of data that differ in quality (e.g., normalization of naming standards, reconstruction of missing atoms, bond computation)
- Force field interface (AMBER implementation)
- Structure mapping and minimization algorithms
Our library is complemented by a visualization tool, BiochemicalVisualization.jl, and both packages can be run in Jupyter Notebooks, enabling rapid application development of structure-based bioinformatics applications.
_________________
T 02
CryoCrane
Presenter: Dr. Jakob Ruickoldt (jakob.ruickoldt@uni-potsdam.de)
Authors:
Dr. Jakob Ruickoldt¹; Prof. Dr. Petra Wendler
¹ Potsdam Universität
Screening of cryo-EM samples is essential for the generation of high-resolution cryo-EM structures. Often, it is cumbersome to correlate the appearance of specific grid squares and micrograph quality. Here, CryoCrane (Correlate atlas and exposures), a visualization tool for cryo-EM screening data, is presented. It aims to provide an intuitive way to visualize micrographs and to speed up data analysis.
_________________
T 03
Between sampling and scoring – a fair comparison of self-supervised machine learning methods
Presenter: Prof. Dr. Clara Schoeder (clara.schoeder@medizin.uni-leipzig.de)
Authors:
Prof. Dr. Clara Schoeder; Moritz Ertelt; Prof. Dr. Jens Meiler
Leipzig University
Machine learning (ML) is changing the world of computational protein design, with data-driven methods surpassing biophysical-based methods in experimental success rates. However, they are most often reported as case studies, lack integration and standardization across platforms, and are therefore hard to compare objectively. In this study, we established a streamlined and diverse toolbox for methods that predict amino acid probabilities inside the Rosetta software framework that allows for the side-by-side comparison of these models. Subsequently, existing protein fitness landscapes were used to benchmark novel self-supervised machine learning methods in realistic protein design settings. We focused on the traditional problems of protein sequence design: sampling and scoring. A major finding of our study is that novel ML approaches are better at purging the sampling space from deleterious mutations. Nevertheless, scoring resulting mutations without model fine-tuning showed no clear improvement over scoring with Rosetta. This study fills an important gap in the field and allows for the first time a comprehensive head-to-head comparison of different ML and biophysical methods. We conclude that ML currently acts as a complement to, rather than a replacement for, biophysical methods in protein design.
_________________
Rainer-Rudolph-Awards Session at the Mosbacher Kolloquium 2024
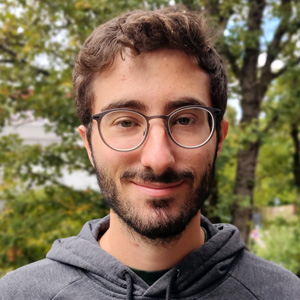
The Arsenal of a Bacterial Predator Revealed by Cryo-Electron Microscopy
Davide Amendola
ETH Zürich
Ixotrophy is a bacterial predation strategy observed in a subset of multicellular, filamentous bacteria of the phylum Bacteroidota and is characterized by their ability to catch prey cells on their surface - like flies on flypaper - and subsequently lyse them to supplement their own nutritional needs. Accordingly, it has been proposed to be an elegant solution to the problem of low nutrient availability in aquatic environments, as well as an important mechanism regulating the dynamics of various microbial communities. The exact mechanisms that mediate ixotrophy, however, have remained largely elusive.
Here, we employed a state-of-the-art multi-scale imaging workflow to visualize and identify the macromolecular protein complexes involved in ixotrophy. We combined cryo-electron tomography and subtomogram averaging with single-particle cryo-electron microscopy to determine the structure of a novel grappling hook-like adhesin responsible for the catching of flagellated prey. By determining the primary amino acid sequence directly from the high-resolution density maps, we identified grappling hooks as the heptameric assembly of a single giant protein. Further, we used various bioinformatic analyses to show that its N-terminal domain resembles other bacterial adhesins, whereas its C-terminal domain directs secretion to the outer membrane via the Type IX Secretion System.
Rainer-Rudolph-Awards Session at the Mosbacher Kolloquium 2024
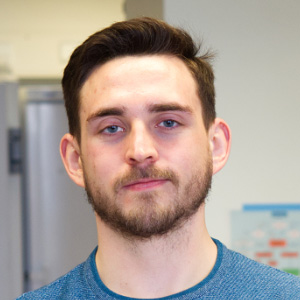
Designing triosephosphate isomerases using generative language models
Alexander Braun
University Bayreuth
Enzymes are wonderful biocatalysts that increase reaction rates by several orders of magnitude. The ability to catalyze reactions in aqueous solution at atmospheric pressure and at ambient temperatures makes these biomolecules an environmentally friendly and cost-effective alternative to synthetic catalysts used in industry. However, enzymes are mostly restricted to reactions occurring in the context of cellular life. The ability to design tailor-made enzymes is therefore of great interest for biotechnology and chemical industry. Methods to create proteins that catalyze a desired reaction have been mostly based on physics-based heuristics or built on improving promiscuous activity in natural proteins. These methodologies are highly time-consuming and in need of extensive screening. As a shift of paradigm, recent successes in the application of language models based on the transformer architecture in protein sciences inspired the development of unconditional and conditional language models, such as ZymCTRL, to design new protein sequences. Being trained on the BRENDA database of enzymes, ZymCTRL generates putative enzyme sequences according to the enzyme commission number used as input. We assess experimentally the performance of this language model to generate triosephosphate isomerases (TIM), an obligatory oligomeric well-researched enzyme class catalyzing its reaction near the diffusion limit. Shallow filtering of generated putative enzyme sequences resulted in three out of twelve de novo TIMs being active in vivo and able to complement a TIM deficient E. coli strain. In depth characterization of the best performing
artificial enzyme shows in vitro activity just two orders of magnitude below its natural counterparts. Based on the results we propose a filtering mechanism to increase experimental success rates of artificially generated proteins even further. This study highlights the potential of protein language models as tools for the generation of tailored enzymes directly from sequence.
Rainer-Rudolph-Awards Session at the Mosbacher Kolloquium 2024
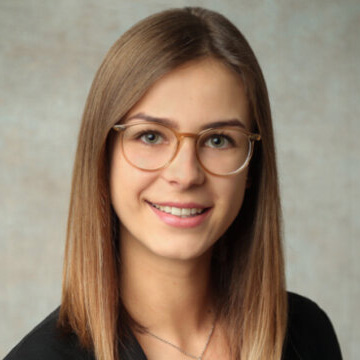
Engineering of single domain antibody-based Bispecifics
Britta Lipinski
Merck KGaA, Darmstadt
Bispecific antibodies offer a targeted approach for cancer treatments by simultaneously engaging two different antigens. The ability to recruit and conditionally activate a targeted immune cell population enhances specificity and efficacy while minimising off-target effects in the absence of the tumour-associated antigen. The use of camelid-derived single-domain antibodies (VHHs) offer several advantages, such as their small size and high stability, but more importantly, in the context of complex multi-specific antibodies, they allow the possibility of multiple ‘plug-and-play’ reformatting options and easy combination with Fab-based paratopes, as they do not require an additional light chain. The main master thesis project focuses on the generation of potent NKCE (natural killer cell engagers) formats that bridge NKp46 on NK cells with EGFR on tumor cells to redirect NK cell cytotoxicity against EGFR-positive tumor cells (Lipinski et al 2023, NKp46-specific single domain antibodies enable facile engineering of various potent NK cell engager formats). In an additional project, single domain antibodies were used as surrogate agonists to mimic the cytokine functionality of IL-18 (Lipinski et al 2023, Generation and engineering of potent single domain antibody-based bispecific IL-18 mimetics resistant to IL-18BP decoy receptor inhibition.). For both projects the impact of valencies and the spatial orientation of individual paratopes within the overall design architecture were further explored by antibody engineering to augment the desired functionality.
Abstracts
Abstracts
Exploring antibody repertoires using deep language models
Presenter: Lea Brönnimann (lea.broennimann@unibe.ch)
Authors:
Lea Brönnimann; Chiara Rodella; Thomas Lemmin
University of Bern, Faculty of Medicine
The rapid advancement of deep learning has extended into the biomedical field, enabling scientists to leverage these powerful methods to analyze large biological datasets. Among these datasets, collections of antibodies have grown significantly in recent years, with the aim of advancing therapeutic design. Antibodies are crucial to adaptive immunity, with diversity arising from heavy-light chain pairing and somatic hypermutation. To better understand the architecture of antibody repertoires, we trained deep learning models to investigate the sequence and pairing dynamics of human antibodies.
An in-depth analysis of the latent space embeddings learned by our language models revealed biologically meaningful clusters, including distinctions based on gene families and B-cell differentiation states. To explore the interdependence of heavy and light chains, we trained machine translation models to generate light chain sequences from heavy chain inputs. While achieving moderate average sequence identity with the true paired light chains, we observed distinct modes within the sequence identity distribution, potentially reflecting biological patterns of heavy-light chain compatibility.
These findings highlight the utility of deep learning models in antibody repertoire analysis, providing tools to study gene usage, maturation, and pairing. This approach offers a powerful framework for understanding antibody diversity and could inform the development of therapeutic antibodies.
Abstracts
Abstracts
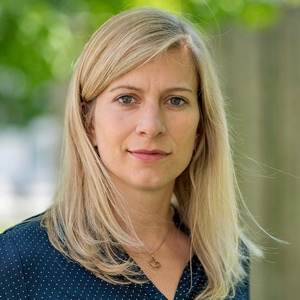
Hybrid AI and Open Source for Drug Design
Andrea Volkamer
Data Driven Drug Design, Saarland University, Saarbrücken [DE]
Addressing the complexities of drug design – exemplified here on human protein kinases – necessitates innovative approaches that blend artificial intelligence (AI) with domain expertise. With over 6,000 human kinase structures available in the PDB and around 70 small molecule kinase inhibitors, persistent challenges such as drug promiscuity, resistance, and unexplored kinase territories remain.
This presentation showcases open-source approaches that integrate domain knowledge into AI frameworks to overcome data scarcity issues and enhance model generalization. Leveraging openly available kinase data, we demonstrate how hybrid AI and classical methods can generate new insights and foster community engagement. The TeachOpenCADD platform [1,2] serves as a versatile tool for orchestrating diverse computer-aided drug design (CADD) tasks, exemplified on individual kinases. We introduce freely available resources to support kinase research and incorporate structural data to enhance prediction accuracy and guide drug discovery efforts. These resources include active learning on the KinFragLib kinase fragment library for inhibitor design [3], and structure-based deep learning approaches for affinity prediction [4].
These projects highlight how hybrid AI approaches fuse experimental with computational insights to advance the frontier of kinase-focused drug discovery.
References:
[1] S. Dominique, et al., TeachOpenCADD 2022: Open Source and FAIR Python Pipelines to Assist in Structural Bioinformatics and Cheminformatics Research. Nucleic Acids Research, 2022. https://doi.org/10.1093/nar/gkac267
[2] M. Backenköhler, et al., TeachOpenCADD goes Deep Learning: Open-source Teaching Platform Exploring Molecular DL Applications. ChemRxiv, 2023. https://doi.org/10.26434/chemrxiv-2023-kz1pb
[3] S. Dominique, et al., KinFragLib: Exploring the Kinase Inhibitor Space Using Subpocket-Focused Fragmentation and Recombination. Journal of Chemical Information and Modeling, 2020. https://doi.org/10.1021/acs.jcim.0c00839
[4] M. Backenköhler, J. Groß, V. Wolf, A. Volkamer, Guided docking as a data generation approach facilitates structure-based machine learning on kinases. Journal of Chemical Information and Modeling, 2024, 64, 10, 4009–4020 https://pubs.acs.org/doi/10.1021/acs.jcim.4c00055
Abstracts
Integrating geometric deep learning with explainable artificial intelligence to assist protein engineering
Presenter: Dr. David Medina-Ortiz PhD (dmedina@ipb-halle.de)
Authors:
Dr. David Medina-Ortiz PhD; Dr. Mehdi D. Davari PhD
Leibniz Institute of Plant Biochemistry (IPB)
Designing proteins with desirable properties has many applications in biotechnology. Traditional protein engineering approaches have benefited from advancements in artificial intelligence (AI), yet integrating AI into protein engineering introduces challenges in explainability and interpretability. Explainable Artificial Intelligence (XAI) addresses these challenges by providing insights into predictive model decision-making, identifying critical amino acid residues, uncovering physicochemical properties, and guiding protein design strategies transparently.
This work presents an XAI-based framework for protein design, integrating geometric deep learning, transformers, and XAI. Predictive models are developed using graph neural networks and embeddings from pre-trained protein language models. Graph-based methods, attention mechanisms, and feature engineering detect key residues and properties, enabling explanation of model predictions. These insights guide targeted modifications to enhance protein fitness by linking predictions to key residues and properties.
Validated on datasets like GB1 and TrpB, the framework identifies critical residues and properties that can improve protein fitness. The integration of generative strategies based on diffusion models is also being explored for E(3)-equivariant protein generation. This framework accelerates the design process and advances AI-driven protein engineering by enhancing interpretability, reliability, and predictive accuracy.
Abstracts
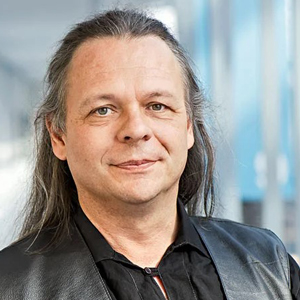
Artificial Intelligence deciphers the code of life written in proteins?
Burkhard Rost
Technical University of Munich, School of Computation, Information and Technology, München [DE]
The objective of our group is to predict aspects of protein function and structure from sequence. The wealth of evolutionary information available through comparing the whole bio-diversity of species makes such an ambitious goal achievable. Our particular niche is the combination of evolutionary information (EI) with machine learning (ML) and artificial intelligence (AI). 30 years ago, the marriage of machine learning and evolutionary information (in the form of Multiple Sequence Alignments) allowed a breakthrough in secondary structure prediction. The same principle has been underlying all state-of-the-art predictions of protein structure and function and is also the root for the program that broke through in protein structure prediction, namely AlphaFold2.
Over the last two years, it has become possible to deep learn the language of life written in proteins through protein Language Models (pLMs). The information extracted is transfer learned to supervise learn protein prediction with annotations. I will present three particular new methods predicting protein structure (1D: secondary structure, membrane regions, & disorder, 2D: inter-residue distances/contacts, 3D: co-ordinates) and protein function (sub-cellular location, binding residues, GO terms), and the effects of sequence variation using pLMs. These embeddings allow for some applications to reach for others to surpass the state-of-the-art without using evolutionary information.
Crucial in all of this is the understanding of the AI and the control of database bias. For both computational biology could serve as a sandbox to prepare more sensitive applications of AI in society.
Abstracts
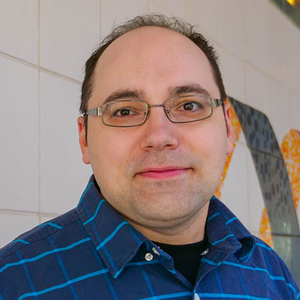
Inverting Protein Structure Prediction Models for Protein Design
Sergey Ovchinnikov
/Cambridge [USA]
For this talk I'll describe some recent advances in inverting protein structure prediction models for protein design, contrasting them to more recent diffusion based methods. I'll go through some examples of designing cyclic peptides, large new folds and protein binders.
Abstracts
Abstracts
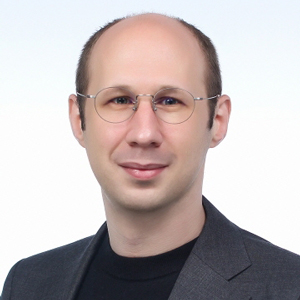
Supercharged Protein Analysis in the Era of Accurate Structure Prediction
Martin Steinegger
/Seoul [KR]
Protein analysis has been transformed by machine-learning methods, with highly accurate structure prediction tools like AlphaFold2 and ESMFold leading the way. These methods have generated an unprecedented number of publicly available protein structures, with the AlphaFold database and ESMatlas now containing over 214 and 620 million predicted structures, respectively. To utilize this wealth of structural data, we have developed advanced tools like Foldseek, Foldseek-multimer and FoldMason to efficiently search and analyze these massive datasets. Additionally, our BFVD database significantly improves viral protein structure predictions by leveraging homology searches across petabases of sequencing data. This expanding landscape of structural information is revolutionizing genomic and proteomic annotations. In this talk, I will explore how these new tools and resources are enabling researchers to uncover novel biological insights and accelerate discovery across diverse fields of biology.
Abstracts
Learning conformational ensembles of proteins based on backbone geometry
Presenter: Leif Seute (leif.seute@h-its.org)
Authors:
Nicolas Wolf¹; Leif Seute²; Vsevolod Viliuga³; Simon Wagner⁴; Jan Stühmer²; Frauke Gräter¹
¹ Max Planck for Polymer Research; ² Heidelberg Institute for Theoretical Studies (HITS gGmbH); ³ SciLifeLab; ⁴ IWR, Heidelberg University
Deep generative models have recently been proposed for sampling protein conformations from the Boltzmann distribution, as an alternative to often prohibitively expensive Molecular Dynamics simulations. However, current state-of-the-art approaches rely on fine-tuning pre-trained folding models and evolutionary sequence information, limiting their applicability and efficiency, and introducing potential biases. In this work, we propose a flow matching model for sampling protein conformations based solely on backbone geometry. We introduce a geometric encoding of the backbone equilibrium structure as input and propose to condition not only the flow but also the prior distribution on the respective equilibrium structure, eliminating the need for evolutionary information. The resulting model is orders of magnitudes faster than current state-of-the-art approaches at comparable accuracy and can be trained from scratch in a few GPU days. In our experiments, we demonstrate that the proposed model achieves competitive performance with reduced inference time, across not only an established benchmark of naturally occurring proteins but also de novo proteins, for which evolutionary information is scarce.
Abstracts
Bayer Pharmaceuticals PhD Award
Evolution of a protein fractal
Franziska Sendker
Dana Farber Cancer Institute, Boston MA 02215, USA, franziska.sendker@mpi-marburg.mpg.de
Abstract not submitted yet
sponsored by:
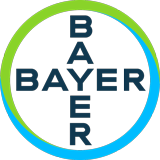
Abstracts
Karl Lohmann Prize
Structural Investigation of the Relaxed Cardiac Sarcomere by Electron Cryo-Tomography
Davide Tamborrini
Basel Univeristy, Biocenter, <a>davide.tamborriniunibasch</a>
The sarcomere is the basic unit of striated muscles and consists of interdigitating thick and thin filaments. The two types of filaments slide on each other, resulting in the shortening of sarcomere itself, thereby generating work. The thin filament comprises filamentous actin (F-actin), troponin (Tn), and tropomyosin (Tm). The thick filament is the force-bearing part of the sarcomere, and it comprises myosin, titin, and myosin-binding protein C (MyBP-C). The vast majority of the mutations responsible for familial hypertrophic cardiomyopathy and other heart and muscle diseases are borne by components of the thick filaments. However, despite its central importance, it remained unclear how thick filaments are structurally organized and how its components interact with each other and with thin filaments to enable highly regulated muscle contraction in the cardiac tissue.
During my PhD, I aimed to elucidate the molecular organization of the thick and thin filaments in the relaxed state. I resorted to cryo-focused ion beam milling (cryo-FIB milling) and cryo-electron tomography (cryo-ET) to investigate the molecular architecture of the native mammalian cardiac sarcomeres.
The reconstruction of the thick filament reveals the three-dimensional organization of myosin, titin and myosin-binding protein C (MyBP-C), clarifying the structural basis of muscle contraction at sub-nanometer resolution. These results provide a molecular blueprint of the cardiac sarcomere and pave the way to forthcoming research aiming to explore muscle disorders involving sarcomeric structural components.
Abstracts
Abstracts
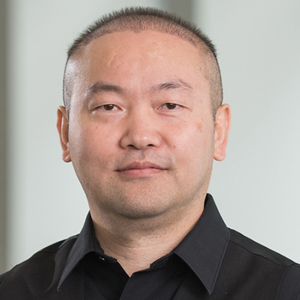
Towards an AI-based solution of protein structure prediction problem
Yang Zhang
Department of Computer Science at School of Computing; Department of Biochemistry at Yong Loo Lin School of Medicine; Cancer Science Institute of Singapore, National University of Singapore
The past decade has witnessed revolutionary changes in computer-based protein structure prediction, mainly driven by the artificial intelligence (AI) and deep neural-network learning techniques. In this talk, we discuss structure prediction results in recent community-wide CASP experiments, showing that new deep-learning approaches, built on coevolution data from multiple sequence alignments, can result in consistent and successful folding of large proteins with complicated topologies. Of note, AlphaFold2 trained through end-to-end transformer networks could fold nearly all protein domains in CASP14 with 2/3 of them having accuracy comparable to low-resolution experimental solutions. In the most recent CASP experiments, new advancements over AlphaFold2 have been made by integrating end-to-end learning and protein language models with fragment-assembly simulations. These achievements essentially break through the 50-years-old barrier of homology-based modelling and marked a solution, at least at the fold-level, to the single-domain protein structure prediction problem. Nevertheless, constructions of atomic-resolution models for multi-domain and higher-order quaternary protein structures remain challenging. Furthermore, the black-box nature of AI-based approaches remains a barrier to unravelling protein folding dynamics. Given the power of AI and rapid advancement of the field, it is expected that these challenges should be addressed in a foreseeable future by coupling deep learning techniques and metagenome sequencing databases, with the aid of advanced structure assembly simulation algorithms.
Abstracts
New Machine Learning-Driven Approaches for Peptide Probe and Drug Discovery
Presenter: Arman Simonyan (gtk897@ku.dk)
Authors:
Arman Simonyan; Prof. Wouter Boomsma PhD; Prof. David Gloriam PhD
Copenhagen University
Two-thirds of human hormones act through ~800 G protein-coupled receptors (GPCRs). The vast majority (71%) of these hormones are peptides or proteins, which also account for an increasing share of drugs [1]. The study of peptide-receptor recognition is thus essential for understanding physiology, pathology and for drug design.
Our work aims to address the modeling problem of peptide-receptor recognition by leveraging AI-based methods and unique data from the field hub GPCRdb [2]. We benchmark the state-of-the-art in silico peptide design protocols, including RfDiffusion [3], AlfaFold Hallucination [4], ProteinMPNN [5], ESM-IF1 [6], Frame2seq [7], AFinitialGuess [8], on our dataset. In addition, we build in-house predictive statistical and deep leaning models representing residue interaction networks across the receptor-peptide interface. The models are trained on a unique data representation, storing data for individual residues rather than the overall protein. This allows peptide data to be inferred across conserved residues in different receptors – enabling use on receptors not targetable with classical methods.
The in silico discovered probes are being tested in vitro by pharmacological collaborators. In all, this will let us discover novel drugs and engineer new probes, enabling functional characterization of understudied receptors that cannot be targeted with current techniques.
Abstracts
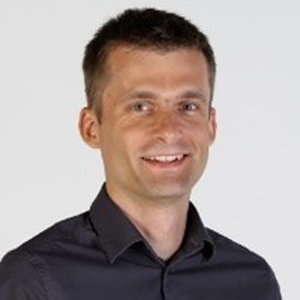
AI-based integrative structural modeling
Jan Kosinski
EMBL Hamburg [DE]
Macromolecular assemblies are essential to biological processes, yet their structural characterization remains challenging due to their size and complexity. Integrative structural modeling addresses this by combining data from various experimental techniques, including electron microscopy, X-ray crystallography, and crosslinking mass spectrometry, with computational approaches. In this presentation, I will discuss our recent advancements in integrative modeling of macromolecular assemblies, emphasizing the incorporation of AI-based structure prediction tools such as AlphaFold. We have utilized these tools to construct a detailed structural model of the human nuclear pore complex (NPC). The NPC, a 120-megadalton assembly regulating nucleocytoplasmic transport, has posed significant challenges for structure determination due to its size and flexibility. By integrating cryo-electron tomography data with AlphaFold predictions, we achieved a comprehensive model covering approximately 90% of the structured scaffold. Additionally, I will present AlphaPulldown, a Python package designed for high-throughput modeling of protein–protein interactions using AlphaFold-Multimer. This tool streamlines the screening process and facilitates the modeling of higher-order oligomers. Furthermore, I will present AF3x, a method that enhances structural modeling by incorporating crosslinking mass spectrometry restraints in explicit atomic representation to drive AlphaFold 3 predictions. This approach can sometimes improve the accuracy of AlphaFold 3 models, particularly for complexes with limited evolutionary data.
Abstracts
Abstracts
AI-based protein design and validation
Presenter: Sophie Binder (sobind@mailbox.org)
Authors:
Sophie Binder¹; Bianca Broske²; Benjamin A. McEnroe²; Marie Kleinert²; Dominic Ferber¹; Julia Meßmer²; Elisabeth Tan²; Tim N. Kempchen²; Caroline I. Fandrey³; Alexander Hoch³; Peter Konopka³; Katja Blumenstock³; Prof. Dr. Matthias Geyer¹; Prof. Dr. Jonathan Schmid-Burgk³; Dr. Stephan Menzel⁴; Dr. Gregor Hagelueken¹; Prof. Dr. Michael Hoelzel²
¹ Institute of Structural Biology, University of Bonn; ² Institute of Experimental Oncology, University of Bonn; ³ Institute of Clinical Chemistry and Clinical Pharmacology, University of Bonn; ⁴ Core Facility Nanobodies, University of Bonn
AI-based prediction of protein structures is a very recent and rapidly evolving topic with high potential in the field of cancer research and development of novel therapeutic agents, in particular in settings of difficult antibody or nanobody production.
Using alpha-fold-based prediction algorithms, we generated libraries of artificial proteins designed to bind specific target structures. Through a cellular screening system, we identified a subset of binders that were validated to successfully bind their respective targets. Binding to target proteins was verified in FACS experiments using cellular systems as well as recombinantly expressed proteins. When studying the binding kinetics using SPR spectroscopy, we observed high binding affinities in the nanomolar range. Further, the AI-Binders exhibited a high thermal stability and a notable ability to refold after denaturation.
The AI-Binders are readily expressed in E. coli and can be easily purified to homogeneity. Preliminary crystallization trials revealed that the AI-Binders are highly inclined to form crystals, enabling their use as crystallization chaperones. By site-specific biotinylation and functionalization with streptavidin, we generated tetravalent target-binding scaffolds, which are promising tools for target protein structure determination using cryo-electron microscopy.
Abstracts
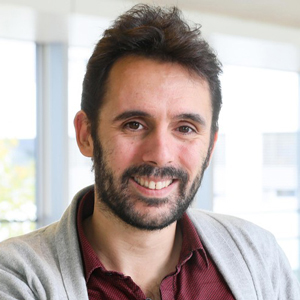
Exploring the Structural and Functional Landscape of Proteins by Computational Design
Bruno Correia
/Lausanne [CH]
The machine learning tool box has revolutionized our ability to design novel molecular entities (e.g. proteins) well beyond what the natural repertoire has explored. Despite the incredible advances, the de novo generation of functional molecules in biological concepts remains an incredible challenge.
In this talk, I will present some of the efforts in our group in designing both proteins and small molecules. Particularly emphasizing different modalities of molecular representation and the interplay with generative ML to facilitate the exploration of unimaginably large spaces of possibilities. Importantly, many ML-based approaches for molecular design fall short in terms of generalization and sampling off the learned distribution. I will present some ideas on how representation can help to overcome some of these limitations.
Finally, I will present some of the approaches developed in our group to embed function into the designer proteins and how we are using these components in cellular systems to control the output of these complex biological devices.
Abstracts
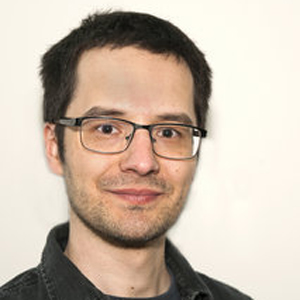
What can we learn from incorrect AlphaFold2 models? (the case of signal transduction)
Stanislaw Dunin-Horkawicz
/Tübingen [DE]
Prokaryotic signal transduction proteins are based on a fibrous coiled-coil backbone in which functional domains can be identified. These domains are specialized for functions such as signal recognition, transduction across the membrane, modulation, and initiation of downstream effects. Experimental structures of these domains have been essential for exploring hypotheses about signaling mechanisms, underscoring the potential of AlphaFold2 to further our understanding. In this work, we applied AlphaFold2 to a peculiar family of signaling proteins that contain long arrays of signal modulation domains (HAMPs), which typically occur in a single copy in canonical signal transduction proteins. Our results suggest that the function of these proteins may depend on the intrinsic conflict between the conformations of adjacent HAMP domains, while also highlighting the applicability of AlphaFold2 to the study of dynamic proteins.
Abstracts
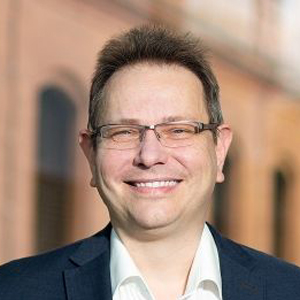
How artificial intelligence is reshaping protein structure prediction and therapeutic design – from small molecules to new modalities
Jens Meiler
/Leipzig [DE]
AlphaFold is revolutionizing protein structure prediction. Not because of its increased accuracy in comparison to the best predictions of prior methods, but because of consistent accuracy across all folded, well-structured proteins. I will discuss some of the remaining challenges such as predicting all biologically relevant conformations of flexible membrane proteins including transporters, ion channels, or receptors.
With the availability of highly accurate structural models for most proteins, structure based drug discovery is experiencing a renaissance. The availability of large 'make-on-demand' compound libraries coupled with computational ultra-large library screening fundamentally changes the paradigm in (academic) probe and drug development projects to 'in silico' first! I will introduce these concepts and detail several new algorithms to accomplish these tasks.
While AlphaFold is close to a golden bullet for protein structure prediction, computational design of protein and pepti detherapeuti ccandidates is much more challenging even with the use of artificial intelligence. One challenge is that the desired goal is functi on, the design algorithm focuses on structure implying that it might have the target function. A second challenge is the inclusion of chemical space with limited training data such as non-natural amino acids. I will give an overview of several new algorithms developed by us and others combined with illustrative applications.
Abstracts
De novo design of multiple-geometry forming protein scaffolds
Presenter: Anna Backeberg (Anna.Backeberg@campus.lmu.de)
Authors:
Anna Backeberg; Elizaveta Maltseva; Ho Yeung Chim; Prof. Dr. Alena Khmelinskaia
Polyhedral protein assemblies are highly functional materials with applications in areas such as drug-delivery or vaccine development. While the de novo design of static protein nanoparticles with one specific target geometry is well established and exceedingly accurate, this study aims at the design of rigid protein monomers that can simultaneously self-assemble into different geometries. Just like having a versatile ingredient in a kitchen pantry, our goal is to establish "off-the-shelf" programmability of protein assemblies with a set of universal building blocks, facilitating the targeted design of new and functional protein nanoparticles. Combining current Artificial Intelligence (AI)-based design methods with physics-based approaches, we established a pipeline for the design of such multiple-geometry forming protein scaffolds. Our pipeline tackles this challenge on two levels: (i) the generation of backbones matching multiple geometries; and (ii) the design of degenerate sequences compatible with equal formation of the target architectures. Our approach paves the way for a new generation of highly versatile protein building blocks.
Abstracts
Training-free framework to design protein-based binders
Presenter: Dr. Kateryna Maksymenko (kmaksymenko@tuebingen.mpg.de)
Authors:
Dr. Kateryna Maksymenko¹; Prof. Julia Skokowa; Prof. Andrei Lupas; Dr. Mohammad ElGamacy
¹ Max Planck Institute for Biology
Designing specific and high-affinity protein binders remains an attractive yet challenging goal. Recently, the combination of restrained diffusion, message-passing neural networks, and structure prediction models has shown significant improvements in binder design. However, as deep learning tools keep advancing, they remain constrained by the structural and binding patterns learnt from natural complexes. Therefore, we sought to develop a training-free solution to the binder design problem.
Our complementarity-centric framework first identifies suitable design scaffolds and binding poses utilizing an analytical surface fingerprinting method. Next, the selected scaffold is mutated to carve a binding site de novo. Sequence design is carried out using the in-house developed Damietta software (Grin et al., NAR, 2024), which employs tensorized energy calculations. Finally, design candidates undergo rigorous in silico validation through accelerated molecular dynamics.
We applied this framework to design binders against two oncogenic targets: vascular endothelial growth factor and interleukin-7 receptor-α. Experimental characterization of a small set of designed proteins (ranging from 2 to 8 per target) identified nanomolar binders for each target site. By relying on first principles, our generalizable framework is well-suited for handling ligands that are underrepresented in the PDB, and we are currently adapting it to design protein-based binders for small-molecule targets.
Abstracts
Abstracts
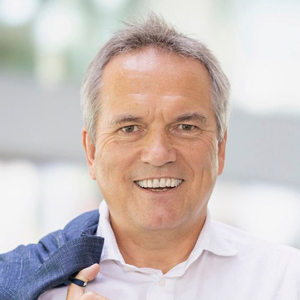
Otto Warburg Medal
From Otto Warburg to Riboregulation: a 100 year journey in metabolism
Matthias Hentze
European Molecular Biology Laboratory (EMBL), Heidelberg
RBPs) enjoy almost half a century of emerging recognition for their widespread functions in posttranscriptional gene regulation. Thanks to Otto Warburg and his colleagues, metabolic enzymes and pathways have been researched over a 100 year journey, and the paths of metabolic enzymes and RBPs have rarely crossed. Iron regulatory protein 1 (IRP1, formerly IRE-BP), identical with cytosolic aconitase (ACO1) was an early and notable exception (Constable et al., 1992).
Following the discovery that the RNA-binding proteome is far larger than previously anticipated (Castello et al., 2012; Hentze et al., 2018), riboregulation, the direct control of protein function by RNA, has begun to emerge as a new paradigm of biological control (Horos et al., 2019; Huppertz et al., 2022; Chatterjee et al., 2024) that adds a surprising twist to our understanding of metabolic enzymes and metabolism. We are beginning to understand molecular mechanisms of riboregulation, and I will discuss their implications for metabolism, cell biology and disease mechanisms as well as the arising new therapeutic opportunities.
Constable, A., S. Quick, N.K. Gray and M.W. Hentze. Modulation of the RNA-binding activity of a regulatory protein [IRE-BP] by iron in vitro: switching between enzymatic and genetic function? Proc. Natl. Acad. Sci. U.S.A. 89, 4554-4558, 1992.
Castello, A., B. Fischer, K. Schuschke, R. Horos, B.M. Beckmann, C. Strein, N.E. Davey, D.T. Humphreys, T. Preiss, L.M. Steinmetz, J. Krijgsveld and M.W. Hentze. Insights into RNA biology from an atlas of mammalian mRNA-binding proteins. Cell 149, 1393-1406, 2012.
Hentze, M.W., A. Castello, T. Schwarzl and T. Preiss, A brave new world of RNA-binding proteins. Nature Rev. Mol. Cell Biol. 19, 327-341, 2018.
Horos, R., M. Büscher, R. Kleinendorst, A.-M. Alleaume, A.K. Tarafder, T. Schwarzl, D. Dziuba, C. Tischer, E.M. Zielonka, A. Adak, A. Castello, W. Huber, C. Sachse and M.W. Hentze, The small non-coding vault RNA1-1 acts as a riboregulator of autophagy. Cell 176, 1054-1067, 2019.
Huppertz, I., J.I. Perez-Perri, P. Mantas, T. Sekaran, T. Schwarzl, F. Russo, D. Ferring-Appel, L. Dimitrova-Paternoga, E. Kafkia, J. Hennig, P.A. Neveu, K. Patil and M.W. Hentze. Riboregulation of Enolase 1 Activity Controls Glycolysis and Embryonic Stem Cell Differentiation. Mol. Cell 82, 2666-2680, 2022.
Chatterjee, A., M. Noble, T. Sekaran, V. Ravi, D. Ferring-Appel, T. Schwarzl, R. Rampelt and M.W. Hentze. RNA promotes mitochondrial import of F1-ATP synthase subunit alpha (ATP5A1). doi: https://doi.org/10.1101/2024.08.19.608659.
sponsored by:
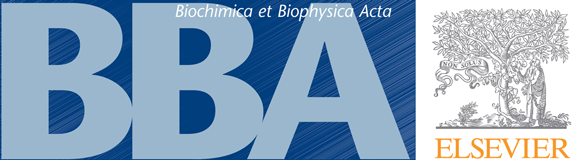
Abstracts
Abstracts
A novel rotamer library for nucleic acids enables the design of protein-nucleic acid complexes with the protein design software MUMBO
Presenter: Marharyta Makarova (marharyta.makarova@fau.de)
Authors:
Marharyta Makarova¹; Dr. Martin T. Stiebritz¹; Prof. Dr. Beatrix Suess²; Prof. Dr. Yves A. Muller¹
¹ FAU Erlangen-Nuremberg; ² Technical University Darmstadt
Current AI-tools for designing macromolecules have been struggling with DNA and RNA structure prediction and design, as the amount of respective experimental data available for training is significantly lower than that of proteins. Therefore, developing alternative approaches remains of interest. MUMBO is a program that allows designing protein-protein interactions and protein-ligand binding pockets by means of side-chain packing algorithms, where alternative amino acids and their rotamers are generated from libraries on a fixed backbone and the most favourable ones are selected based on the lowest overall energy. In order to extend MUMBO capabilities to designing nucleic acids, a nucleotide rotamer library is needed. Based on statistical analysis of pseudorotational angles and dihedral angles of more than 175,000 nucleotides from experimental structures deposited with the PDB database, a discrete pseudorotational angle-dependent nucleotide-specific rotamer library was derived. We validated the library by rebuilding more than 20,000 nucleotides in a custom dataset and showed that it allows modelling nucleotides with accuracies even slightly better than for amino acids. The implementation of the novel nucleotide rotamer library in MUMBO enables modelling and designing DNA and RNA sequences on a fixed backbone together with protein-nucleic acid interaction interfaces.
Abstracts
Abstracts
Abstracts
Title to be announced
N.N.
not submitted yet
Abstracts
In Silico Design of Enzymes to Degrade Short-chain Per- and Polyfluoroalkyl Substances (PFAS)
Presenter: Salomé Guilbert PhD (salome.guilbert@epfl.ch)
Authors:
Salomé Guilbert PhD¹; Prof. Philippe Schwaller²; Prof. Ursula Roethlisberger²
¹ Ecole Polytechnique Fédérale Lausanne; ² EPFL
Increasing concern over the persistence and toxicity of per- and polyfluoroalkyl substances (PFAS) has pushed for the need to design technologies for their remediation. To date, the only commercially degradation method involves costly and unsustainable thermal deconstruction. Bioremediation stands as an attractive, cost-effective and sustainable solution for degrading such pollutants, although few bacteria and no enzymes are known to achieve the full degradation of PFAS.
The in silico rational design of novel enzymes for PFAS degradation first involved the selection of a suitable biological target that had the potential to be optimised for better substrate binding, catalytic activity and thermal stability. A theozyme was created with the catalytic residues of fluoroacetate dehalogenase, a well-studied enzyme capable of breaking the strong carbon-fluorine bond (460 kJ/mol) in fluoroacetate. First, RFDiffusionAA helped generate a library of suitable scaffolds around the theozyme. After sequence design with ProteinMPNN, AlphaFold was used to filter the sequences that folded in the desired structures.
To assess the catalytic reaction of these enzyme designs, QM/MM simulations will be performed. Further, selected biomimetic systems will be optimised with genetic algorithms, classical molecular simulations and machine learning tools such as AI.Zymes. This process will be applied to short-chain PFAS molecules, often overlooked as degradation products of longer-chain PFAS.
Abstracts
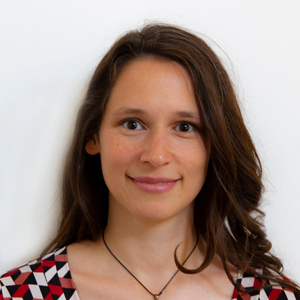
The potential of AI for experiments in structural biology
Andrea Thorn
/Hamburg [DE]
Structural biology is key to understanding basic processes of life and a major driver for the development of new therapies. However, these structures do not directly result from the experiment, but are merely models which explain the observed data according to a priori knowledge. Consequently, our structures are only as good as our limited understanding of the underlying principles.
However, in crystallography, the gap between model and reality remains clearly evident in hard to interpret maps and large R values. In cryo-EM, model properties do not align well with the actual specimen. Machine learning methods, in particular convolutional neural networks, have been applied to a variety of problems in the electron cryo microscopic and crystallographic structure solution of biological macromolecules. However, until recently, their acceptance by the community was limited to tasks where they replaced repetitive work and visual checks were easy, such as particle picking, crystal centering or crystal recognition. With Artificial Intelligence (AI) based protein fold prediction now revolutionizing the field, it is clear that their scope could be much wider, including structure determination, prediction and validation. Experimental biomolecular structure determination could potentially profit immensely from AI, which may even pave the way to joint analyses of data from different labs and methods, which would significantly advance our understanding of the molecules that govern key biological processes.
Whether we will be able to exploit this potential fully will depend on the manner in which we use machine learning: training data must be well-formulated, methods need to utilize appropriate architectures, and outputs must be critically assessed, which may even require explaining AI decisions.
Abstracts
Publishing Workshop
How to publish your research in scholarly journals
an interactive session with Prof. Lienhard Schmitz, Executive Editor of Biochimica et Biophysica Acta – Molecular Cell Research and Laura Mesquita, Executive Publisher of the Biochimica et Biophysica Acta journal family, Elsevier
In this interactive workshop, Executive Editor of BBA Molecular Cell Research, Prof. Lienhard Schmitz, and Executive Publisher of the BBA family of journals, Laura Mesquita, will present on how to prepare your research for publication. From tips on converting your thesis into a publishable research article, to everything you need to do before you hit “submit” in order to avoid a desk rejection, to navigating the peer review process efficiently for a successful outcome.
Date/Location:
Friday, March 21, 12:00 - 13:30
Malzboden
Registration (max. 125): https://terminplaner6.dfn.de/de/b/7d4a2a71f7e38aa4246139ac17b446c5-1115115
Industrial Workshop
Demonstrating eLabFTW: A Free and Open-Source Electronic Laboratory Notebook
In this session, we will explore eLabFTW, an open-source Electronic Laboratory Notebook (ELN) designed to meet the diverse needs of research groups across a range of disciplines, including chemistry, biology, physics, and more. eLabFTW provides secure storage and management of scientific data, ensuring the integrity, confidentiality, and probity of results through advanced features such as qualified timestamping, cryptographic signatures, and comprehensive audit logs.
Adopted by leading research institutions like the CNRS, Institut Curie, Vrije Universiteit Brussel, and IRD, eLabFTW has undergone rigorous security audits and risk analyses. The platform offers flexible deployment options, including SecNumCloud-qualified secure hosting, as well as local installation for complete control.
During this demonstration, attendees will learn how to use eLabFTW and explore customization options to enhance their research workflow. We will discuss the laboratory inventory system, scheduler, and other features. We will also consider ways in which eLabFTW can support the goals of the open science movement via the .ELN file format, encourage compliance with data integrity standards, and facilitate the generation of FAIR data. Join us to learn how this free and open-source solution can transform the way research labs manage and protect their scientific data.
Speaker:
Sydney Koke (Sydney@deltablot.email, Deltablot)
Time/Location:
Thursday, March 20, 13:30-14:00
Lecture hall
Funding Workshop
Funding Opportunities for Graduates in Life Sciences
This talk is aimed at Master and PhD Students as well as early Post-Docs in Life Sciences. Elaborating on the funding opportunities of the German Research Foundation (DFG) it will provide early career researchers an overview of respective possibilities to kick-off a career in academia. A Program Director from the DFG will be available for your questions.
Speaker: Nikolai Raffler, DFG
Time/Location:
Friday, March 21, 12:00 - 13:00
Tenne
Funding Workshop
Research funding at the Klaus Tschira Foundation
Do you know the Klaus Tschira Foundation?
We fund natural sciences, mathematics and computer science – with a focus on research, education and science communication.
In this session, we will focus on research funding. We support outstanding and creative ideas in basic research that have the potential to significantly advance science.
In detail, you will learn about
- What we fund
- Requirements for funding
- What specific funding lines are available
- What other types of funding are available for researchers
- Information on the application process and deadlines
...and much more.
The session is aimed at all scientists from post-doc level upwards.
Information on funding can also be found on our website https://klaus-tschira-stiftung.de/en/funding/
Speaker:
Anke Lischeid, Program Manager Research, Klaus Tschira Stiftung, Heidelberg
Time/Location
Friday, March 21, 13:45-14:30
Darre
Registration (max. 55): https://terminplaner6.dfn.de/de/b/82142a4733a87d5e45cf4843e9acee37-1115151
Industrial Workshop
Empowering Cryo-EM workflows with AI and CryoFlow
Industrial Workshop by Thermo Fisher Scientific
Empowering Cryo-EM workflows with AI and CryoFlow
Smart EPU and Tomography 5 / Tomo Live represent cutting-edge advancements in electron microscopy, incorporating AI-driven capabilities to enhance Single Particle Analysis (SPA) and electron tomography workflows, respectively.
Smart EPU utilizes advanced machine learning algorithms to facilitate routine tasks such as the selection and classification of squares and foil holes in cryo-EM grids. Using predefined criteria, the best areas for acquisition can be determined, and robust automated imaging, on-the-fly particle selection, 2D alignment, and 3D density map reconstruction can be performed in a fully automated manner for comprehensive sample screening and increased output of high-resolution structures.
Tomography 5 / Tomo Live are used for tomography workflows. Tomography 5 assists the user in setting up tomography runs, including performing beam shifts with predictive tracking for multi-shot tomogram acquisition within a single tilt sequence. During acquisition, Tomo Live automatically handles motion correction of acquired frames, alignment of tilt series, and real-time reconstruction using SIRT for enhanced productivity, intuitive data quality assessment, and faster visualization.
These enhanced capabilities for SPA and tomography are enabled by CryoFlow, an integration platform common to both workflows. Results are displayed in a web-based platform for increased accessibility, enabling users to efficiently review acquired data and analyze a variety of quality metrics, such as CTF confidence range, alignment accuracy, and astigmatism, among others.
Together, these AI-powered tools significantly enhance the field of electron microscopy, offering researchers effective solutions for detailed structural analyses at the molecular level.
Speaker:
Javier Fernández Collado /Thermo Fisher Scientific
Time/Location:
Thursday, March 20, 13:00-14:00
Tenne
Registration (max. 80): https://terminplaner6.dfn.de/de/b/de5d8d074969cb32345c576fe990b67b-1115138
Introduction to the Rainer-Rudolph-Awards Session at the Mosbacher Kolloquium 2024
The Rainer Rudolph Foundation promotes and supports basic and applied research in protein biochemistry and biotechnology. Every year awards are given to young scientists who have made outstanding advances in these fields. In this session, the awardees of the Rainer Rudolph Prize will present their work in short lectures.
Davide Amendola /Zürich [DE]
The Arsenal of a Bacterial Predator Revealed by Cryo-Electron Microscopy
Alexander Braun /Bayreuth [DE]
Designing triosephosphate isomerases using generative language models
Britta Lipinski /Darmstadt [DE]
Engineering of single domain antibody-based Bispecifics
Panel Discussion: Big Data, Big Challenges - How do we manage research data?
The GBM working group “Young Investigators” invites you to a workshop on “Big Data, Big Challenges - How do we manage research data?”.
Do you want to organize and store your research data efficiently?
Do you wonder how to ensure the reproducibility and integrity of your data?
Do you ask yourself how to comply with data management policies and best practices?
Join us to explore these questions and more with experts from both academia and industry, and learn how to manage your research data effectively!
Panel participants:
Nikolas Carpi, elabFTW
Sydney Koke, elabFTW
Michael Jendrusch, EMBL Heidelberg
Kersten Rabe / KIT
Thursday, March 20, 2025, 12:15-13:30 (Lecture Hall)
Open for all conference participants.
We kindly ask to register at https://terminplaner6.dfn.de/de/b/875e1f733f1d9bc8c4b5a0bdbaa75e50-1115050
Meet the Prof
Meet the Prof
(For students only)
Once again the Junior GBM will organize the established event „Meet the Prof“ with distinguished speakers from science and research.
In an informal atmosphere you will get the chance to ask the questions that keep you up at night - e.g. how do I make a career in science? Did the speaker have fun on his/her scientific path and which experiences were decisive?
You are welcome to join and ask further questions.
Thursday, March 20, 12:00 - 13:00 (Room: Darre)
Chair: Katharina Bachschwöller
Guest: Tanja Kortemme
Registration is required.
Friday, March 21, 12:00 - 13:00 (Room: Darre)
Chair: Philip Müller
Guest: Matthias Hentze
Registration is required.
The number of participants is limited. A registration is required on https://terminplaner6.dfn.de/de/b/ef1628a1d2dfc35c4d428964274cda29-1115010
Meet the industrial Scientist
Meet the industrial Scientist
(For students only)
Analogous to the well-known format "Meet the Prof", this is about research in the pharmaceutical industry.
What are the differences between industrial and university research? How do the ways of thinking, approaches and focal points differ? Other topics include publications, patents, job security and career opportunities in a pharmaceutical environment compared to universities.
Guest: Alexander Rives (EvolutionaryScale)
Chair: Jens Martin
Date/Location:
Thursday, March 20, 12:00 - 12:45 /Tenne
The number of participants is limited. A registration is required on https://terminplaner6.dfn.de/de/b/ef1628a1d2dfc35c4d428964274cda29-1115010